Ingestion to Insights
50 min video / 39 minute readSpeakers
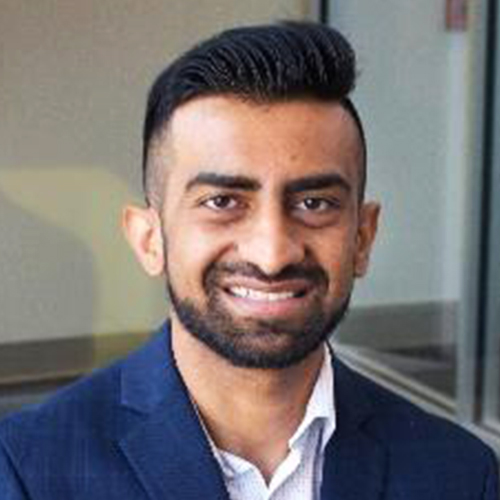
Nadir Khoja
Solutions Architect
Feyen Zylstra
In this informative session, attendees will learn how a manufacturer – or any automation setting – can successfully begin their industry 4.0 journey. Starting with data collection, then moving to data visualization, alerting, and analytics, Ignition allows organizations to do it all. And, with multiple web-based architectural options, Ignition offers flexibility while keeping cyber security in mind.
Transcript:
00:16
Chaz Cooper: I'm Chaz Cooper from Inductive Automation. I'm a Technical Analyst, and I'm here to... I'm gonna be here as the moderator for today, and this is going to be “Ingestion to Insights,” and I'll be introducing Nadir from... A Solutions Architect. He started his North American manufacturing journey at the leading international manufacturer of die sets and steel plates while pursuing his master's in electrical engineering at the University of Windsor, Ontario. Nadir went on to teach data analytics to postgraduate students at St. Clare College. Currently, with Feyen Zylstra, he helps enable manufacturers to achieve their Digital Transformation goals and implement Industry 4.0 technologies across organizations. And here's Nadir.
01:13
Nadir Khoja: Thank you. Can you guys hear me?
01:16
Audience Member 1: Yeah.
01:16
Nadir Khoja: Perfect. Thank you so much for the kind words, Chaz.
01:20
Chaz Cooper: Welcome.
01:20
Nadir Khoja: So I guess you guys wanna know what we're here for, topic called “Ingestion to Insights.” Before we move on to “Ingestion to Insights," I'm just gonna share something about me, which will help us paint a picture, tell the story properly. I started my journey in manufacturing as a estimator/continuous improvement personnel. And we were just making... Thanks, Chaz... We were looking at steel plates, getting some chunks taken out of them, putting it together, selling them, and then shipping it to the customer. I really, really, really quickly realized that data was becoming a very, very, very crucial part because everybody had questions. When something goes wrong, there are questions. There are a lot of different fingers pointing, "This could be the reason, that could be the reason," but nobody had facts.
02:19
Nadir Khoja: So, I started diving deep into what could potentially answer these questions, and the answer relied into the data, but how do we get to that data? How do we get some insights out of that data? So when I was asked by Inductive Automation to potentially, maybe able to speak here, I was like, well, what, the whole industry, it really doesn't matter in what you do, I know we're here for Ignition and manufacturing, but everything is becoming towards database systems, like social media. It could be your houses and water bill, whatever. It's all getting… moved towards cloud and becoming a data point for something. Today's agenda would be… I'll share with you what Feyen Zylstra does and what I do at Feyen Zylstra. We're going to talk about data is the “new” oil. We're gonna talk about conventional setup of what somebody who's a manufacturer, starting their Industry 4.0 journey, what base solution would look like, what a potential solution should be, in comparison.
03:45
Nadir Khoja: We're going to talk about the data science approach because, like you guys heard, I also teach data analytics at a postgraduate college in Windsor. And that's exactly why I'm here because everything's becoming data-oriented solutions. We'll see how that applies to manufacturing. And then obviously, why Ignition is going to be something that we all want and could use to cater your solution towards an Industry 4.0-based IoT platform. And at the end, we'll have a lot of questions, hopefully.
04:23
Nadir Khoja: Alright. So something about Feyen Zylstra, I, basically, am going to thank my marketing department who put this together, but oops... Yeah. If you have visited a hospital, if you've fed your dog, if you've eaten ice cream somewhere, if you've taken a shower, which I hope everybody does almost every day, if you've flown on a plane, which some of you did coming here, and if you've eaten turkey at a Thanksgiving dinner, if you're driving to school, and if you vacuum your house regularly, we've basically, Feyen Zylstra, have basically touched you in some or the other way.
04:57
Nadir Khoja: We're basically a 600-plus people company. We're growing rapidly as well. But let's talk about more... And so we have different branches, but the branch that I work for as a solutions architect is called Industrial Tech. And Industrial Tech basically plays on all five levels of manufacturing. You start from level zero, which would be your basic work doers, your robots, your control panels, your PLCs, your machines. We have an army of people who could do PLC integrations and SCADA systems and get your data out from those machines to you, and all the way up to enterprise level. So we actually have all the support that could basically, if you have no data collection, we could take you from level zero to level five. But the main point we're going to talk about today is level three, Information Systems. That's what we're here for. Let's dive into the data, right? Data is the "new" oil, and I put quotes around it. How many of you agree that data is the “new” oil? Two?
06:10
Audience Member 2: Absolutely.
06:11
Nadir Khoja: Sorry?
06:12
Audience Member 2: Absolutely.
06:14
Nadir Khoja: Absolutely. I would kind of try to make you think a little differently. Yes, it is the new oil. So was the actual oil when we found it. It was always in existence. So was data. We have paintings in caves that people looked at and said, okay, this is some information. What do I get out of this? So data has always been in existence, but humans have never had those tools to extract that information and then do something about it. Like we looked at the paintings in the caves. It told some kind of a story that meant that we were lighting fire to cook our meal or stay away from an alligator. They're not your friend, but you may want to have a dog by you. We've found these evidences from the cave paintings. So the point is, we're going to actually think about the tools that we have now. Now, I'm not gonna go dive deeper into computational power and cloud services and all that because that's a different topic in itself.
07:24
Nadir Khoja: But nowadays, we have so much available tools that you don't have to develop. You can just go subscribe to a service which will enable you to take your data, push it into that service, and you get your analytical solutions deployed within sometimes days, sometimes weeks. The whole industry, the manufacturing industry, has started to change how they look at a problem, how they look at solving something that has been a pain for so many years, and that's having facts and/or feelings. We all have seen, as integrators, we have seen, in meeting rooms, we've seen people that are with the company for 25 years, and they have tremendous knowledge. And those people are looked upon and asked, "Okay, based on your experience, what do you feel is happening? Or what do you think is happening?" Feel and think. These are the two words.
08:18
Nadir Khoja: Yes, that person's very important. That person's feelings and his knowledge or her knowledge and experience is very important, but that is still a feeling. You need facts to be able to confirm that feeling. And people are moving towards that direction a lot. Humanity is now creating data as fast as we're blinking. Actually, we're creating way faster data than we're even blinking. So now, enough of history on data, let's see how manufacturers have their data collected in whatever way.
08:56
Nadir Khoja: So manufacturing execution system. Yes, we've all heard about it. That's a source of data for manufacturers. We have assets management system... Asset, sorry. And then we have inventory management system, then we'll have material handling systems. And not all of these would apply to a certain company or organization, but some of it would. We have industrial control systems, we have tool control systems, we'll have process control, inspection systems, sales and customer management, and accounting and financial systems, and many more.
09:31
Nadir Khoja: So, just based on a very quick list that I could think of, I put together, how many data sources can an organization have? Now, when you want to start your data collection and ingestion, and by the word “ingestion” here, I mean how do you digest that data and how do you make sense out of it, where do you start? You basically don't really know what to do with all this... Which systems you're gonna look for. So that's why we're here. Now, let's say we have a pilot to do for Industry 4.0. We have... I'm running a company, there's four people in the company, which are my managers, and then there's a team underneath them.
10:14
Nadir Khoja: And they would say, "I like Industry 4.0. I wanna see dashboards. Let's go. Let's do something." So what you would do is typically a pilot would be, let's do a proof of concept. Let's start with connecting our machine and equipment, start data collection and visualization. Sure, let's do it on one line, which has five machines and one main PLC that is going to send all the data out, so I can control everything from the one PLC. And so, assuming it has 500 tags, which is relatively a very, very low number. We've been into places where we've heard about that one line has 42,000 tags and 32,000 tags, which was basically three processes. But no, I tried to stay conservative. I know this is a liberal state, but still.
11:07
Nadir Khoja: Some tags... So let's assume some tags generate a continuous signal every second. So the volume of data that you're gonna look for... Oh, and by the way, Ignition has this architecture beautifully provided to anybody who does not know how to start, so you have your pilot, you looked at Ignition, and you said, "Yes, let me get Ignition. I won't even buy the license yet. I'm just going to do a trial." Perfect.
11:33
Nadir Khoja: Now if you had one tag that's generating a signal every second, that's going to exponentially increase the data points that you're going to start collecting on your tags. And the reason that I put it out there is because Ignition has the historical configuration settings on the Tag Historian, that what you would do is you would just enable it, yes or no. Should I collect history in this tag, yes or no? And, of course, you wanna see your data later on to connect the history. And there are some very, very, very important things, which is the deadbands and some kind of a filter that you could apply right there, which is fantastic. But I'm gonna show you how that is also not enough sometimes. Okay?
12:21
Nadir Khoja: So I think if you wanna do deadbands, yearly how much data you'll collect is way out of proportion because then when you try to... How many times people have asked questions? How did we do last year? And you hit your report and you wait 55 minutes before that report does anything for you. So that's where we don't want to be. And the reason the reports take so long is because if you configure stuff this way, your relationships in the database will look something like this. And to a lot of us here, this might look... Yeah, it's okay. I'm just connecting the relationship between one table to another. Well, I'm gonna debate, and I'm gonna show you why this is not okay because let's understand that these are multiple sources of truth, that we're trying to chase a truth. We're trying to answer a question. These are multiple sources of that truth, or it has some parts that it will be giving a hint from this table to another table, but you gotta combine it.
13:29
Nadir Khoja: The reporting, after you did all the data collection, let's say you connected your equipments through Inductive Automation's fabulous modules and all that, you got your Historian turned on. So you're collecting data, and you made your visualization. So now let's go back to our story of four floor managers and a lot of people are gonna meet them. The guy was like, "Hey, you wanted me to do a pilot. Here's the pilot, here's the report I made. Fantastic." But do we all stop there? No. We actually start asking more questions. How did we do last year? Like I just said. Well, okay, I don't know how to answer that, but let's see. Why did we perform the way we did last year? Whatever the question was. What's our OEE? What's our quality? And many more.
14:23
Nadir Khoja: I've seen people, and I can't take names, but I've seen organizations where we asked them, "How do you know there's a problem?" And the answer we got back was, "There's a big horn in the back. It just goes off. That means there's a problem." So, when I heard that, this was basically me in my mind...
14:48
Nadir Khoja: That yes, you should not start by, "Yes, I want to do a pilot system. I want to do this." You quickly got up, went, and started deploying stuff, and now once you did your first report, that was the visualization. More questions came in. But you can't answer those questions because the report tells you nothing. I've seen people do a pilot with seven machines. It worked really well. But then, once that is expanded to within the plant, nothing worked after that.
15:19
Nadir Khoja: Because, like I said, you just turned on the Historian, you're collecting data, and it was to a point where the system was not even able to respond. Everything was just frozen because the data collection was so heavy it was not thought through. So, a conventional setup would look like what I just showed you in terms of what Inductive Automation had as a simple architecture. But at Feyen Zylstra, as a solution architect, what I try to do and what we, as a team, try to do is we're not gonna bring you a solution and tell you how to use it. We're going to come to you and ask you what you need. And based on my expertise, we tell you, "This is probably what you need." And along the lines of to this presentation, I'll explain why Ignition is also one of the best options that you can use. So if you take a solution and try to fit it into the problem, this is probably what it would look like. Yes.
16:18
Nadir Khoja: In the meantime, there'd probably be a guy by your doorstep selling you a hedge trimmer but do not want to pay for it because you already own a lawn mower. And that's a lot of things... That's a lot of times we were seeing in the industry we already have a person who likes to do Python in-house. He does it on his own time. We already have a person who's taken Raspberry Pis and done a lot of projects around the house, so why don't we use him? That's great. That person probably has a lot more information that you need on your project, but you still need to invest in the right tools when you have to.
16:56
Nadir Khoja: Okay. So what a solution should actually be. Now at Feyen Zylstra, we're probably trying people to use the acronym RUMSS. It's trying to just make sure that you follow the right steps to be able to pick a solution. Or even designing a system. So the first one is Repeatable. Like I said, there was a customer who did it, did a pilot with seven machines, but when you got scale, nothing worked. So Repeatable. We want it to be Upgradeable. We're also running into, not Ignition, but other companies where they had the system running back in 2016, and they turned it back on in 2022. Nothing works 'cause it was running on Windows. It was running on something in the background. Windows upgraded, but the system wasn't designed to be upgradeable. We also want things to be Modular, which Ignition is. We want it to be very, very modular. We want it to be Scalable, and we want it to be Secure. So if you have your solution catered around these five RUMSS rules, you'd never go wrong. And also, you would never have a false start to your project.
18:20
Nadir Khoja: So the main point about me doing this session is the data science approach. Like I said, the whole system is, every solution that you're going to implement in any manufacturing facility or outside of it is going to revolve around data. And as a data analyst, and when I teach my students, what we always talk about is you need to have a very holistic approach, and you need to start asking the right questions. So, the data science approach is, if you are doing a machine learning project or if you're doing data cleaning for a large government data set, where, let's say, the city of New York has bicycle accidents all over the city, how do we show it as a visualization? Okay.
19:11
Nadir Khoja: First, you need to understand what are you trying to get out of it, which is defining the question. If you cannot define the question of the solution that you're trying to look for, then there's no point in doing all this. So this applies directly to our manufacturing solutions that we're trying to implement as system innovators, as manufacturers. If you don't know what you're trying to chase, then you can't just, so the example that I showed about doing a pilot, yes, we were doing Industry 4.0, ran with it, did a great little visualization, but after that, we're dead in the water. So before you even go think about Googling what Industry 4.0 software we should have, this should be step one, define what your question is.
20:08
Nadir Khoja: Then, the data collection, also, we're still not doing any kind of implementation. Now we're gonna discuss about the data collection. So, in data science, there's like multiple versions of truth, which is first-party data, second-party data, third-party data. First party is exactly what you own inside your own organization. I'm not gonna go into second and third party because second party is similar data that you also have, but collected by somebody else. So let's say, if you're thinking Coca-Cola and Pepsi, they both do the same thing. Coca-Cola's first-party data is their data, Coca-Cola's second-party data is Pepsi's data. So sometimes you need to collect that as well so that you could compare if you're doing something right or wrong or if you're trying to chase a question, which we'll have more training and insights on that side.
20:51
Nadir Khoja: Third-party data is basically customer feedback in forms and stuff like that, but we only care about first-party data. Once you decide what type of data you want to collect based on the questions you've asked, then we would go, “How do we clean this data?” Meaning, “How do we prep this data?” And now, data prepping is the most time-consuming and the most, I would say, effort that you'll put into the project when it comes to data. And it is exactly what Ignition helps you to do. And I'll show you how.
21:30
Nadir Khoja: So, once you've asked the question, okay, what is the question? What are we trying to chase? It could be multiple questions. It could be, “I wanna know how my labor hours are being utilized.” Efficiency. “I wanna know how we did this quarter. I wanna know how we did two quarters in a row. What is happening with a new machine in production line?” It could be multiple questions, but each question has to go through these steps of define the question. What data you need for it? So the data collection. How would you store the data? Which is data cleaning.
22:05
Nadir Khoja: And then what would you need to do to get the insights out of it, which is what type of machine learning or what type of analytical solution you wanna put onto it. So, if some of you have not heard about diagnostic, 'cause usually people hear about descriptive, which is identifies what has already happened. Something already happened. We go and see what happens, so it will tell you through this analytical solution. Then we have diagnostic. Diagnostic focuses on understanding why something has happened, right? Then we have predictive, which is it allows you to stop something before it happened. And then prescriptive allows you to make a recommendation for the future, that how do I not let this happen again? Alright.
23:00
Nadir Khoja: So the last part is sharing the results. Now, this part is very crucial. So you've answered your question, what has to be done? What problem we're trying to solve, what type of data would support that problem, then we're going to what type of data cleaning and how we would store it, then you would go to what type of analytical way we should approach it, it could be statistical, you don't even have to go this far, or it could be machine learning and AI.
23:26
Nadir Khoja: Then once the results come out, machine learning and AI would just spit out a Boolean, true or false, or sometimes it'll spit out a reading. It'll be a two-digit, three-digit reading with two decimal, three decimal points. What does that mean? So for you to be able to transfer the results of your solution via a very good visualization, that's also very important. And again, we're still not implementing anything. We're just going to make sure that we have all these steps listed out first before we go in, try and invest company money into a tool.
24:04
Nadir Khoja: So, why Ignition? Now when I said in the earlier slides where people were doing their pilots, first step is to collect information and connect everything, from the equipments and from your platform system. After you defined your question, you went through the data science approach. Now you exactly know that if I want to understand how my new machine is doing, well, I only need to go collect that new machine's data. I don't need to connect the whole line. So if the question was defined properly, you're not gonna connect the whole line if the question was just about that one machine.
24:47
Nadir Khoja: And I know this is very, very obvious, but data science is actually very interesting to me because it is a list of obvious things that we should do before we would approach a solution. And let me think of something else. Let's say you wanted to understand, “Why did we have two hours of downtime last week?” And the question was, “Okay, I want to understand downtime for this particular line or plant,” or whatever. So then you would definitely put yourself in those shoes and try to understand, okay, “What does downtime mean?” Well, these 15 tags, which tells me downtime relates to this.
25:32
Nadir Khoja: You're just gonna cater your solution to that, and you're only going to collect 15 tags for that question to be answered. And, move it up the chain, go to visualization, do your analysis, and you see, yes, we had downtime because the machine had low fuel or low levels of whatever, but that would not have been easier to do if you just went and collected every single point from your machine, every level from your machine and everything the machine offers because how many times you've gotten a new machine that you're connecting and it has all the tags that you can pull out?
26:04
Nadir Khoja: Once you pull it out, well, what do you do with that? So then you start moving down to the granular level saying, okay, I have 15 tags pulled out, but I only need two, so we're trying to go over first, we're trying to first put everything down, pen and paper and understand what we need to collect, then we use the strategy. So coming back to, once answering, answering all the questions, we connect our machines and equipment to the system using Ignition's fantastic modules: MQTT, OPC UA, and they're always coming up with better ways to connect.
26:41
Nadir Khoja: Then we have data collection modules that also Ignition has, which is SQL Bridge and Tag Historian. Then we have for visualization. We have reporting modules and Vision/Perspective charts. Now, we're going to actually focus on data collection. And because this is all about touching how data science approach is to a solution in the manufacturing entity in the 21st century, an average data scientist or anybody who is working with data will spend 60% of their time just cleaning the data. So what I'm gonna talk about is how do you do data cleaning with the help of Ignition as soon as you connect those tags into Ignition?
27:26
Nadir Khoja: So let's say we have 500 tags, back to our pilot, and everybody has heard about our database, right? We all have databases in one way or another. It could be an Excel sheet, or it could be a cloud-based MongoDB or PostgreSQL or whatever, SQL server or whatever database you have storing your data. But in terms of structuring, how you will collect your database on your question, when you ask for your things, your day-to-day operations have to go into your database because you're going to frequently ping it. Your real-time operations will also go into your database.
28:15
Nadir Khoja: Then you'll come to the data warehouse, where historical reporting will be collected in a way and stored there. Now, I'm not saying that you need separate locations, you need separate databases. It could just be a table, depending on what the solution is. It could be a table. We could call it “database.” There could be another table. We could call it “data warehouse.” But the point is keeping them segregated, siloed out, because you don't want to ping the database for every question you wanna answer. So, if you structure the data the way you wanted to answer your questions like this, it would be much, much easier. And again, you would not wait 55 minutes to run a report.
28:57
Nadir Khoja: Data warehouses are also used for Extraction, Transformation, and Loading. ETL. We call it ETL. Some of you might have heard it, some of you might have never heard it, but ETL is basically my tag was showing me 45 as a reading for five hours. What does that mean? So there was a threshold that I could compare it to, that means I'm transforming it, and I'm going to interpret it into saying, okay, 45 is going below threshold, so that is bad for me. So any type of ETL work that is pulled using reporting, it has to go to data warehousing.
29:40
Nadir Khoja: The last point is data lakes. This is basically your dumping ground. Could be archiving. It could be... So remember I said earlier, when you can turn on the Historian tag enabled, and you can put the filters there, and you could keep it that way? Yes, those can go straight to the data lakes because, yes, you need your historical data. Again, it could be used... It could come useful… It could be handy when you are trying to answer larger questions. Larger questions, which are something like, “Do we need to hire more people? Okay, how did we do last year? Were we working so much overtime? Were the machines operating and we were still working overtime, or were the machines not working, and people were working overtime?” So things like that could be, but those things that you'd need larger data sets, they can go into data lakes. Any kind of archival stuff, you can put in your data lake, but never ever archive or start pushing your tag values into the database for a longer period of data collection. Anything that's in the database and data warehouse has to be cleaned up.
30:47
Nadir Khoja: If I had a line, say, in five years, sometimes depending on your data volume, two years; once two years happen, archive it to the data lake and reset your database. It all depends. It's all about what you need, not what everybody else does. So again, it goes back to the data science approach. Ask the question first, “What do you need?” Now, if you were going to ask the question again, “Yes, I wanna know downtime on my machine, but how far in the past, I want to keep always in a history.”
31:19
Nadir Khoja: So let's say we are 24/7, and we have three months of cycles of manufacturing your product, then you switch products. So then, yes, you need to know three months' worth of data, and that will go into your database. So everything switches back to question one. Define your question correctly. The more time you take defining the question correctly, the easier it will be for you to develop, deploy, and implement the system.
31:43
Nadir Khoja: So how can you do data collection in a very effortless and easy way using Ignition? Here's the answer. Have you guys heard about Transaction Groups? Yeah. So Transaction Groups are a very, very powerful tool. Transaction Groups have, I think, three things, basic OPC transactions, which enables you to read from the PLC and sometimes write back. It also enables you to run expressions on the values. So like I said, you wanted to transform, right? I have a 45-degree, 45-number reading coming from a tag. What does that mean? So I can compare it to a threshold, and I can store high or low, or incorrect, or green or red, whatever I want already transformed into the database. So I don't have to go to the database, pull the 45, the numeric value, and then pull it into another visualization tool, and then put an expression at the end there saying, if this is 45, it's below this, that means it is low. You could actually transform it right here and store the low or high value or green or red value into the database without even going through the collecting unnecessary data.
32:58
Nadir Khoja: Actually, let's talk about this. So like I said, you can create your relationships. So remember the multiple tables I showed, with the relationships going... Errors going all around? You don't even need that. You'll be able to create a single source of truth based on your question using the Transaction Groups. But by that, what I mean is I don't have to have data into tables and then join the relationships, and then run a query, which will take, again, five, 10 minutes, whatever or depending on what the solution is. You don't have to do that. You can create a logic of imagining multiple tables as multiple tags, make it relationships right there in Transaction Groups, and store the end result of your question that would be answered into the database. So, imagine each question you wanted to answer has its logic written down into the Transaction Groups. It could be as an expression, which is changing, if this, then that.
34:06
Nadir Khoja: You use expressions to understand the values coming in and use those values stored into the database. There's triggered expression. So again, based on your question you ask, if something goes wrong, then I wanna know the level of this tank. If my CNC machine is spinning properly, but I'm still losing tool, so as soon as the tool breaks, okay, the trigger happens, store this this this this into the database, those values. So all I'm saying is, all the relationship that you have to the traditional way of storing data, yes, I have it on the table, could go away if you define your logic correctly, and you only would know what to define if you ask the question first.
34:54
Nadir Khoja: So, I know I'm repeating myself a lot, but asking the right question is the way to go. And sometimes it helps me… It helps me in my relationship, too. 'Cause sometimes asking the right question could avoid your sleeping on the couch or sleeping in the basement. So once you've defined the question, you've collected the right data, you've now taken your Transaction Groups, you've defined the relationship and not gone towards storing everything into the table, you've defined that into the Transaction Groups, and then now you're storing what exactly you want to look for, then comes the visualization. And this is the most important part. This is what people jump to right away. As soon as people say, "Yes, I wanna do Industry 4.0, I wanna see a chart." Yeah, they'll go, “What kind of chart I wanna make?”
35:46
Nadir Khoja: A Gantt chart, a Pareto chart. They would rush to this, but 99% of the time, if they rush to this, they're not going to have the right information displayed on those charts. Ignition has very powerful tools for reporting. And those reports are also… You could email them, you could text them, you could do all sorts of notification things, but it also provides you, because of it being SQL, you could also use that same data because you've all structured it already into Transaction Groups, you're going to then able to use it into third-party apps like Power BI, could be Tableau, it could be just your Excel sheet. Some people are still using Excel. Yes, we've seen them. They all love Excel. That's how I started venturing into analytics.
36:32
Nadir Khoja: So, before I go down into the conclusion, let's look into some common mistakes that people do when they start their Industry 4.0 journey. And I'm not saying I've not done that, but I haven't done all of them. Not learning the basics. What that means is I was given a task, and let's say it's about understanding, “Why did we have a blowout in the section of this line?” So if I was the guy who deployed that line, I'll just go run straight to that problem and try and start at the source. Sometimes it could just be that it was an operator error. So you have to cover your basics. You don't want to assume that, “Yes, I have the expertise. I don't need to talk to anybody. I'm just gonna go.” That happens in a lot of situations.
37:43
Nadir Khoja: Not asking questions. The whole data science approach is ask questions. So you have to ask questions. And when I'm teaching, I tell my students there are no wrong or right questions. There are just questions. I'll decide if they're right or wrong, but you can still ask them. Not learning the business context. You need to define how is the business going to benefit from what you're trying to ask me to do. Whatever relationship you have with your subordinates or your managers, it really doesn't matter. You have to ask them the question saying, "Hey, I know I'm just the controls engineer, but how is the business going to make sense out of this system that we're making or this report that you want me to make?" What is the business context?
38:32
Nadir Khoja: 'Cause it helps you shape your story that you're trying to tell. Not cleaning the data. So if you did not go the Transactional Group route, you're not gonna clean the data. That means you're gonna have GIGO or GIGO, garbage in, garbage out. 'Cause if you don't clean data, it's all garbage. You'll struggle to show the right data point on the chart because there's like 60,000 points. Which one do we show? So data cleaning, again, is the biggest time-consumer, and with Transactional Groups, you are eliminating that right off the bat.
39:08
Nadir Khoja: Rushing to build. My boss told me to make this system a chart. I'm just gonna make a chart in two days. Once that chart is made, it still didn't answer the right question. So do not rush to build the chart. Take the time, ask the right questions, go around. You might have to ask questions to different people, not just your source. Sometimes you need to ask your question to your source. “Yes, you want me to make this thing? I need to work with you a little bit more,” but sometimes you need to involve everybody in the chain. And I think you should involve everybody in the chain so that you don't miss anything.
39:46
Nadir Khoja: Not learning the domain knowledge, which is chasing the highest paid person's opinion, HiPPO. I call it HiPPO because, again, I know, it's a corporate ladder, we all want to make sure that we get our name up there, and when the time comes for promotion or an upgrade, yes, I want to be in the eye. They want to think about me. But when you're doing this project, you cannot only chase those opinions because sometimes, the people who are working on the floor, they have to be involved in every single thing that you're trying to do.
40:28
Nadir Khoja: We go into a company, and they ask, “Okay, well, who do you want to meet?” And we ask them, “We want your... From your plant manager to your maintenance manager to your quality manager, to your engineering manager, and even the guy who's working on the line, just bring them all into one room,” because you may satisfy HiPPOs, but sometimes HiPPOs are just... At the end of the day, it should be a holistic system, helping the business, not satisfying HiPPOs.
41:00
Nadir Khoja: We're all engineers, and we hate documentation, but if you don't do documentation, then you would not learn from your mistakes, so a lot of people never document what they did. I never used to, but now, if you look at my OneNote, it's like a thesis. You should always document. I'm not saying this, write everything you do, but take breaks in your development cycles. If something crucial was answered by somebody in your questioning phase, just record it because the next time you do the same thing, it will help you look for the same type of information before you go build something.
41:45
Nadir Khoja: And the last and foremost thing, this is more towards people's ego. "I know what to do. I'm just going to go and do it. They told me to do it. I'll show them I can do it." And you isolate yourself. Isolating yourself, it should happen, but it should not happen throughout the whole project. Yes, you need to put your head down and do your stuff. You need to isolate yourself, but you cannot do that with data approach, and you cannot do that with designing a system like what we are trying to design for our customers and clients in the manufacturing realm because every day, there could be two same company robots working the same motions, with the same PLC program. But one guy could be picking up a puck, one guy could be picking up a whole truck.
42:34
Nadir Khoja: Their wear and tear, their data points, their stress levels, all that will be recorded differently. So you cannot isolate yourself and say that, "Yeah, I've already done this. I'm going to make sure that I'll come back." And just the whole point of all these mistakes is that we don't want you to set yourself up for failures. So, hoping these mistakes, you've already documented it, so you don't make the same mistakes that I'm trying to avoid here. With the conclusion, I would say approach your projects, which are related to data, as a story. It should have a beginning, it should have a middle, and an end to be able to go to your insights. So, I'm Nadir Khoja, this was my story, and good luck with your stories.
43:35
Chaz Cooper: We're gonna do questions, I guess...
43:37
Nadir Khoja: Yup. Go ahead.
43:37
Audience Member 3: I couldn't help but… You kept going back to the importance of a question. Judging the crowd and most engineers I know, probably a lot of us … what is the answer? 42. And the importance of getting the right question. So I don't think we spend a lot of time, how do we define what the right question is and what are the right ways we ask, because and worse yet, the wrong questions are thought to be the right one.
44:10
Nadir Khoja: No. 100%, I did not spend a little bit more time on defining how do you define a question. That's an art in itself, but thank you for that question. So the answer to that would be, you basically need the right people inside the room when you start defining your question because, let's say you and me are in the room, and we're trying to solve a problem, and I say, "Well, listen, all I need is I'm confident, I need three tags that show me uptime, downtime, and it'll show me the current consumption, and I know what the motor is doing." But on your end, you're like, that's not going to help me because you're talking about just three tags, but what about the torque of the motor? What about the voltage of the motor? Because…
45:04
Nadir Khoja: So, yes, you need the right people in the room so that... It's not a fight, but it has to be an agreed-upon question before you chase the answer to your question. So, like I said, have the right people in the room because then they will have to speak up because they would sense, based on their experience. Going back to what I said about, it's not that people with experience don't matter, but this is when the experience comes up, and they will speak up and say, "Yes, I agree with you, but I also want these and these information." So we define the question correctly, but thank you for asking that question. Yes?
45:37
Audience Member 4: I'm not sure how to say this succinctly, but if you don't know the question upfront, you talked about understanding the question and then defining a data collection strategy around that. What if you're not recording the right data and you have a new question six months from now, and now you're starting over with going on with that data. Do you have some maybe rule of thumb for what's a good amount of data to collect?
46:08
Nadir Khoja: Yes, and that's a very good question, so... Remember the data lake, you're going to turn on history around all your KPIs that you think... And it's easier to go on your ways than not having it. So I know we have 500 tags coming from this machine. The manufacturers gave them all, data lake is the place to just dump them all, and the data lake will have it all. So, a new question comes up. Yeah. I have the data lake. Is it answering it? Maybe there's more cleaning required, but it may answer it. If not, it'll still you start, it'll still make you start at the right foot to start chasing that question different again. Somebody else had a... Yeah, go ahead.
46:53
Chaz Cooper: Saying one more question...
46:53
Nadir Khoja: Oh, one more question? Go ahead, sir. I think you raised your hand.
46:58
Audience Member 5: Yeah. So I have a common problem in that we have customers who decide, "Hey, I've got a PLC out there. It's getting all of these data points. I don't want to... I haven't brought them in before, but hey, let's bring it all in." And I can't convince them that, you know what? You don't need the actual set points because you're not changing them, and you don't need the things that are just minuscule changes. How do you convince a customer that you need to manage the data you're bringing in, in the first place because you're gonna overwhelm your SCADA system?
47:37
Nadir Khoja: Yes. Again, thank you for asking that. Very, very good question. We've seen that, too. Like I said, we've seen a customer that had 32,000 or 42,000 tags, and they're collecting all of them.
47:51
Audience Member 5: Yep.
47:52
Nadir Khoja: You have the procedure. You are setting those set points, following the procedure. So if you already have the procedure, then you don't need to... 'Cause what they're thinking is, when I want to solve a problem with my screen, I wanna know the set point. But what they don't understand is that set point was coming from your procedure itself. So if you have the process engineers and I... This could be a very different conversation, we can talk later, but customers need to realize that sometimes there's redundancy in their information that they were the ones who set that point, so why are you trying to bring it back in?
48:34
Nadir Khoja: So if they understand the concept of redundancy and they almost take 55 minutes to run their report because now they just overwhelmed their system by collecting everything they have, it's not gonna work. So if you tie it back into process, that will help them understand because sometimes, yeah, they don't understand. Like you would see, you would... And this is... We're all humans, right? In general, if you have an outside contractor come in, who's gonna be in that room? You have the top managers who are in that room. So again, they would say, yeah, I wanna see a set point, but the guy who actually wrote the procedure and who is deciding that for this recipe, this is the set point, is not even in that room. So they need to understand that the process that they defined is going to help them make a better and cleaner system. I hope that answers your question.
49:26
Audience Member 5: Yeah.
49:28
Nadir Khoja: Alright, I think we're good to go? Perfect. Thank you so much, guys. Thank you so much for your time.
Want to stay up-to-date with us?
Sign up for our weekly News Feed.