How To Harness Modern MES for AI and Innovation
52 min video / 40 minute readSpeakers
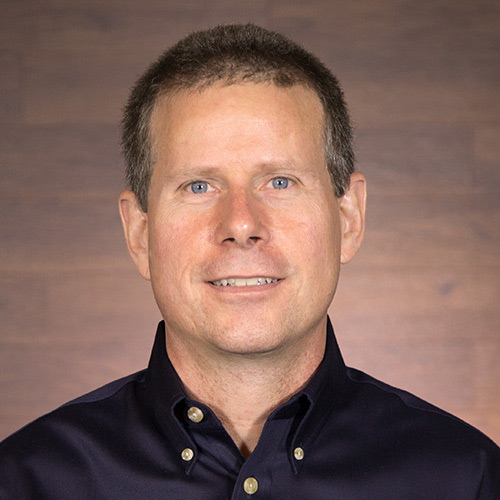
Tom Hechtman
CTO
Sepasoft
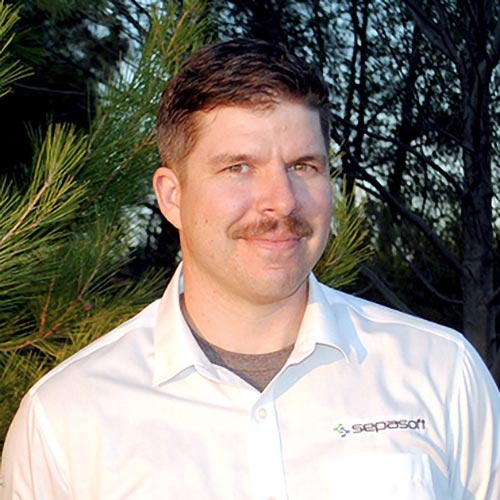
Mark French
Director of Design Consultation
Sepasoft
Learn from MES-experts Sepasoft how MES fuels the success of AI and BI initiatives, driving organizations toward actionable insights and a competitive edge. In the Industry 4.0 era, the success of AI and BI technologies in manufacturing hinges on high-quality data. Manufacturing Execution Systems (MES) play a crucial role integrating with the plant floor and enriching production data with essential metadata, plus adding valuable context for machine learning and advanced analytics. MES provides real-time visibility for informed decision-making and cuts the typical 80% time investment data scientists devote to becoming subject matter experts and preprocessing data.
Transcript:
00:03
Bryson Prince.: Welcome, welcome. Welcome everybody.
00:07
Mark French: I've never been whistled at before.
00:09
Bryson Prince.: I'm Bryson Prince. I'm from our... From Inductive Automation support department. I'm a support software engineer. I'll be the moderator for this session today, and let me welcome you to today's session, How To Harness Modern MES for AI And Innovation. To start things off, let's introduce our two speakers. Today we've got Tom Hechtman, who is the Chief Technology Officer at Sepasoft. Tom Heckman has dedicated decades to work with manufacturers across various industries worldwide. Specializes in implementing production control and tracking systems. In 2010, he founded Sepasoft, focusing on development the Sepasoft MES module suite for Ignition by Inductive Automation. In 2023, Tom transitioned to Chief Technology Officer, where he now drives product innovation and shapes the future of Sepasoft's MES solutions.
01:04
Bryson Prince.: Mark French is the Director of Design consultation at Sepasoft. He leads Sepasoft's Design Consultation Department. His team of sales engineers delivers technical product demonstrations and helps customers and partners achieve success with MES products. Before Sepasoft, Mark worked as a systems integrator implementing control, SCADA, and MES solutions with Inductive Automation and Sepasoft products. Please help me welcome Tom and Mark. Thank you.
01:36
Mark French: Thank you Bryson. Tom, people want to see AI and snuck a slide in here. I think we could do it in one slide.
01:44
Tom Hechtman: Wait a second. We had a whole program. What'd you do to me, Mark?
01:51
Mark French: Just one slide. We know what everybody wants. Everybody wants to use AI to make better pictures of themselves. It's... I think that's what the people want. No? Alright.
02:03
Tom Hechtman: We're done. We can go.
02:07
Mark French: Okay. Joking aside, let's get to our agenda for today. We're gonna do a very brief company update. Now we're gonna dig into digitization and AI, and then we're gonna go over our product roadmap at Sepasoft. Finish it off with some Q&A. So very brief company update. We've been growing additional customers, new industries, many new implementations. So we've been staffing up key strategic staffing addition is of course our new CEO, Tony Nevshemal. Many of you have met him during the conference, so we really appreciate what he's brought to the team. And we're continuing to add staff to the team as well. We've also released guides to enable our users to do more, such as the server sizing and architecture guide, which is a compliment to the inductive guide of the same name, as well as our validation guide for our 21 CFR part 11 customers.
03:09
Tom Hechtman: In addition to that too, we have created some industry specific projects that could be used for demos and starter. So the first two we have are food and beverage, and so it has kitting and mixing and packaging and stuff like that. And the other one's for pharma industry, which really showcases the, you know, validation or verification of, with e-signatures and authentication and work constructions and all that kind of stuff. So we'll be adding to that over time as well. So that kind of reduces the learning curve getting going with our product, so.
03:47
Mark French: And Tom's too humble to mention he's joined the 88 Committee, so you batch nerds know how important that is, so providing some leadership there as well. Yeah, it's worth clapping about. Thank you. Okay. So the heart of the matter. Manufacturing, digitization and AI. So before we get into AI and really leveraging data, I mean, we wanna take a step upstream and talk about why data-driven manufacturing. And I don't think I can do it much better than actually the second day keynote where Travis, Kevin, and Kent showed how automation positively impacts lives, the environment, just people the world over. But real quick, you know, when we optimize operations, right? We run more efficiently where we use less resources, right? We positively impact the bottom line of the company. Just everything, gets better, right? We also have to drive things with data to accomplish compliance and regulations, right? So we need to stay in step with those. At the end of the day, if you're not doing this, you are giving up a competitive advantage in the marketplace.
05:06
Mark French: So really, what is needed to effectively leverage AI and ML in manufacturing? The number one thing that we have seen and we have heard from our community and customers is the need for clean, relevant data. This is so pronounced that we like to throw around the benchmark of about 85% of effort in these projects is expended to get that clean data to AI resources, so...
05:39
Tom Hechtman: In fact, Mark, today I was listening to Bloomberg Radio, and it's not just manufacturing industry where that's an issue, getting the clean data. And that's kind of the phase we're in right now is getting that clean data in many industries. So if Bloomberg says it's true, it must be true.
05:58
Mark French: Well, we also need more than just clean data. We need to know what to do with it, right? So we need expertise with these tools and understanding those tools is not enough, right? We need the domain experience with the manufacturing data. So this problem set mirrors the IT/OT convergence problem that Ignition originally was invented to solve and has been solving, right? We see the same type of kind of two worlds problem there. And we don't know of any companies that don't care about data privacy and costs.
06:32
Tom Hechtman: I haven't found one yet.
06:35
Mark French: So all of these are needed to effectively leverage tools here. Alright. So why hasn't everyone already succeeded in this area? We've got some folks here. There's some islands of success, but this new technology has been a struggle and it's been really expensive as well. The data challenges mirror a lot of the SCADA, HMI and MES challenges that we try to overcome with ignition, right? Poor connectivity, lack of access, replacing manual systems and paper to this day, right?
07:19
Mark French: And I think a big problem is, a lot of folks are believing the hype in the industry, so we wanna to cut through that. One example that a customer asked me not too long ago, they said, we want a system that tells us what data we need to collect. We want the AI to tell us when things are gonna go wrong, and we want the AI to tell us what we need to do to fix those things before they happen. I'm like, okay, I think we might be a little too far on the hype curve there. You know what we believe, but, I think we'll get there, but we're not there yet. The technology's not there yet. So we need to have accurate expectations for what these tools can deliver. And we need to establish delivery of value straight away.
08:07
Mark French: So with that background, that's the problem statement. It's our pleasure to announce SepaIQ. This is a Sepasoft product that we are introducing to the world today, to address these challenges and deliver machine learning and AI value directly to manufacturing today. So as we go through the features of this product and show demonstrations of this product, we want to demonstrate that we can remove barriers, get through the restrictions that are keeping people back, whether that's the technology or the connectivity.
08:42
Mark French: We want to show how this can deliver real time and actionable data to the plant floor immediately, and it can do it without any coding in the implementation. You guys, if you've been with us for any amount of time, you know, we love to write code, we love to let you write code, but we need to have that no code implementation. Don't worry, you can still write code, but you don't have to write code. All right? And we see this product as fulfilling a really key role in feeding other systems. We don't expect to be the only ML and AI service in the world. That would be ridiculous. So we want you to be able to leverage those other guys as well. Tom, do you want to talk about the scalability of this solution?
09:33
Tom Hechtman: Yeah, absolutely. So when you start talking about analytics and where's the best place to do it, you know, having a cluster with multiple servers really is the place to do it. So if you have a... Consider an Ignition system and you're trying to keep track of stuff to the second, and your communications and UIs and all that kind of thing, going and asking for a report for the, you know, last year of data really isn't the system to do it on. Okay? So separating that out is really the answer in having a cluster of servers. So now as you make those requests, you have a bank of servers that can process that and you can keep up with everything else that's going on. And then also just having an environment that is, you know, one configuration and you spin up servers and they automatically get that configuration. One log in, look at the logs, you see the logs for all of those servers in that cluster, or the status and the performance of all those servers in that cluster. So it's just a little easier to administer, sit in the cloud behind the load balancer and is elastic.
10:52
Mark French: So two main reasons we've done this. One, yield faster analytics. Two, feed AI, BI, and ML. So let's talk about how we're gonna do that. First, we're gonna provide AI ready data. To do that, we need effective collection tools such as MQTT, spark plug support, Kafka connectivity, restful API, connectivity to databases supported out of the Box. We'll go through those and much more like 8.3 event streams. And we're not done with that list, right? You, the community are gonna say, Hey, wouldn't it be great if, and you're gonna provide more and say, Hey, we like to use these tools as well. So we're the connectors for those. So we're gonna be adding to that. So we have, you know, easy out of the box data collection capabilities and native communication with Ignition. We also are gonna show here in just a moment how we provide context to that data. This is a key step. We've been hearing about it all week. How do we do this? It's very important. We're gonna show some examples in a moment. We also need to be able to transform that data, normalize it, etcetera. And one of the key things is handling changes. Tom, do you wanna address handling changes? What does that mean?
12:14
Tom Hechtman: Yeah, yeah, absolutely. So if you have a water treatment plant and you're monitoring a pump, pump, speed levels, all that kind of thing, it's pretty straightforward. Or a refinery, pretty straightforward. It's up and running 24/7. And you're just collecting that data and you can start using AI to look at relationships between different values and look at patterns and stuff. But when you get into manufacturing it changes. And I think you folks in manufacturing understand this. Like the lab results come back a day later or an hour later, whatever it is, right?
12:48
Tom Hechtman: And now you have to adjust your yield figures or as simple as a downtime reason, it's changed what originally it was a e-stop and they want to give it more detail and specify that. So in manufacturing data changes. So you need to, when you make that change, echo that all the way up the chain, okay? So if you have a more advanced AI BI system that needs to have the correct data. So, and then, you know, context also is just, you know, it's not time series data. If you have two lots, you need to kind of neutralize the time so that we know when we are manufacturing this lot, that affects running that lot on a different machine. And you can look at those relationships. So when we talk about context, that's what we're talking about in manufacturing.
13:43
Mark French: Excellent. Moving on to analysis. I love our analysis engine, but it is 10 years old, and I think many in this room have explored the edges of the envelope for that tool set. It's a great tool set, right? But it's time for an upgrade to supercharge it, right? So we're gonna demonstrate some performance here today with real time analytics, we really mean that real time part. We want to be able to deliver relevant KPIs from this tool. That could be something like OEE, that could be something else, a different flavor of OEE or whatever KPI you want to calculate. We'll show how to set up those user calculations for the folks that wanna code. We'll show an example of how that's done as well. Of course, you don't have to code to use this.
14:30
Mark French: And then we'll also demonstrate real time predictions, leveraging machine learning models, delivering those values to the plant floor, and talk about how that works with AI as well. So real time predictions, real time analytics. Our last main pillar here is solving the challenges of sharing, right? We've gotta bring the data in, we have to do, you know, that value add work with it, and then we have to share it back. So again, that connectivity upwards towards third party AI. We need to repackage data. Oftentimes, maybe we need to merge data sets and things like that. We need to present that data in the right form for the destination, whether that's, you know, in row or column or some kind of JSON structure or some thing yet to be determined. Yes.
15:23
Tom Hechtman: How about UNS?
15:25
Mark French: Yeah. How about UNS?
15:25
Tom Hechtman: Yeah.
15:27
Mark French: Absolutely.
15:27
Tom Hechtman: Yeah.
15:29
Mark French: And of course, one thing that we see, again, going back to that IT/OT convergence problem that seems to keep popping up, is delivering those data items back to the plant floor. So yes, we wanna go up and we want to positively impact the business and the strategic direction and use the big AI there. But then we also want to leverage that on the plant floor straight away. Okay. It's demo time. So couple comments before I fire up this demo or play the video, right? So these are video recordings from Tom's desktop, so... But this is the only one I'm gonna narrate so you don't have to listen to me too much more. And this is the only one that's sped up. Why is it sped up? Because this is a UI from SepaIQ, which allows you to manage configuration. It's not the main point of the presentation, right?
16:29
Mark French: Okay. So here we are... We're going to edit this group. We're going to enhance the result set with a custom calculation in this case, defined by a script. So as you can see, we're typing in, sped up a little bit. This a single line of JavaScript to define this calculation, right? You may have noticed, adding the column to the group was a drag and drop, you know, visual feature, right? So if you've used custom calculators in our current analysis engine, you know, my rule of thumb is about a hundred lines of Python per data item. That's two orders of magnitude less right there. So that's pretty good. No clapping, that's okay. You guys haven't written as much code, I guess. But I think it's a big deal making this accessible, pretty much to everyone, right? I mean, that's like get value at, with the dataset function in Ignition. So it's that level of programming to provide a custom calculation. I'm pretty excited to see what you guys are gonna do with it. Yeah.
17:43
Tom Hechtman: All right. Thank you. So now we're gonna get in and actually see some performance and some examples here. So this is just OEE. This is nothing new functionality wise, but we include it for a couple reasons here. One is the performance here. So this is actually in a document taking Ignition. You can have a hundred clients all viewing this data. The backend data changes, this updates, you know, it updates the tag. It's pretty straightforward to use, use it with whatever components. So the other thing we do here is ISA-95 is enterprise site area line, kind of that structure. We added in countries and we added in regions in US, but not in Europe. And this is the aggregation of those countries in those regions. So performance is very fast when you flip to that page. Of course, you can present it any way you want to in Ignition, right?
18:44
Tom Hechtman: So we have the sites. You can get your list of sites here and see their values if you don't want to see it graphically. And again, all these were in a document tag, but line is different. Line, when the selection's made here, and this is not sped up, we're going out and getting that data from the SepaIQ server back, so I can't quite read it, but roughly 20,000 lines processed to get those results in roughly a hundred milliseconds or so. So the performance is night and day. It uses streaming and all that. So it's just much, much more performant. And we're actually doing multiple analysis per page. We have our top downtime here, and we also have the OEE figures. All right.
19:32
Mark French: So Tom, I saw in your notes, so I'm cheating. I'm stealing Tom's thunder here. I saw, well, how long did it take to train the model? That's really the question here. So, or not train the model, but to do those calculations, what, there's about 10 million rows worth of data for downtime?
19:52
Tom Hechtman: Yeah. So going back, we've done some, performance, we're in the middle of load testing on this now, but we've done some preliminary stuff and for an example, SPC samples and calculating your X-bar range and all that from your measurements. We go to through 250,000 samples in three seconds, and most of that time is kind of waiting for the database to stream in. We don't allocate memory. It's all using streaming technology. So, built for performance and tested, on that performance and load testing, we're doing mixed load testing. Now, this is, we're take kind of taking a shift here. So that previous one is what did happen or what is happening, right? We're gonna shift into what could possibly happen. Okay? So with this, you can train it on any features. We just happen to have what product it is, the raw material vendor and what the shift is. But you could have any number of features. It's very easy to select those features and say, we want to train against those. Then you put in your target quantity and then this is what we can expect, losses of production today. And so now operations can be proactive and look out for these things. They can put a mechanic out there to watch for something, that kind of thing. So it's really going forward and helping production versus just reporting what's happening now.
21:33
Mark French: So, sorry guys. I jumped again on the slide. So this model was built off of 10 million rows. The...
21:43
Tom Hechtman: Yeah. And...
21:43
Mark French: Yeah, this took about four seconds. Is that right?
21:45
Tom Hechtman: It took about four seconds for it to learn. And you can improve your, at that speed of learning, you can update your learning pretty frequently. The other thing is that's built on, the, we use XG Boost Machine Learning library, that's using that internally if you want to train it outside of the system, and you can import your model in too, so you don't have to do it on the same system.
22:13
Mark French: So we're seeing, results in the, 100 millisecond range here, for live calculations, and we're seeing machine learning model training times, in the single digit seconds. Right?
22:27
Tom Hechtman: Yeah.
22:28
Mark French: So how often do you want to retrain, right? How much do you want to calculate? This is, several orders of magnitude better performance than we've ever thought of. And so, Tom what kind of system spec do you have behind this? You got a warehouse full of Bitcoin mining rigs, or what do you got?
22:47
Tom Hechtman: Yeah, so load, well, we didn't have to put in a power pant nearby. Load testing aside, which we're doing on servers, that was on my desktop, which is six or seven years old, Quad core, who a bunch of apps running. So very high performance, for pretty medium system. So, yeah.
23:12
Mark French: Excellent. So yeah, just, I mean, just next level stuff. So here, take us through another example.
23:20
Tom Hechtman: Yeah. This is an SPC sample. First thing is that chart, it's going, getting all those samples, pretty rapidly. Again, this is a document tag and underlying data changes and updates. But we are also, instead of using SPC rules, we're actually just looking at patterns and we have measurement data that we trained it on and everything here, but you could include other data. So along with your sample data, you can conclude other data. SPC rules don't really support that. So actually quite powerful. And how long have the SPC rules been around?
23:55
Mark French: Longer than you and I.
23:56
Tom Hechtman: Okay. [laughter] So here I put in some sample data based on the previously training, 95% likelihood that that's a defective product. You can do some about it now, put in something more reasonable and we get to like a, somewhere around two, little over 2%. So this is just another way to use prediction machine learning out there. I think there's huge opportunity in this, in the SBC aside base.
24:29
Mark French: Absolutely. So Tom, you showed us, really taking our current analysis to a whole new level, new levels of flexibility with the OEE example and the downtime example. Then you showed us, loss reason, predictions, we talked about model training, and now SPC, all of this data is really, automated really, machine driven typically. What about the human side? What do we have in mind there?
24:57
Tom Hechtman: Good question. Good question. So this is sentiment analysis, or what's the tone of notes that have been typed into the system? So some customers type in notes for downtime or summary at the end of the shift, maybe other maintenance things, whatever. But is the operator getting frustrated? What's the trend there? Are they struggling? Are they optimistic? So that's what this does. And this is a chart showing the sentiment over time. And what's really interesting about this is that take the word supervisor, that's a pretty neutral term just by itself. If you were just doing English language, sentiment of words, okay, but in a manufacturing space, somebody types in supervisor [laughter], it might not be as good. So you actually train these words in the notes against some KPI. Could be downtime, could be loss of production, what have you, and then those words then get your meaning within your industry and your organization. So it's more tailored to your business and your data. We can do sentiment just English language and report that back, but it's not as interesting. And we actually did this. We're, basing this off of notes that we received from a customer of ours. Thank you. Yeah.
26:34
Mark French: I can't see you because of the lights in our eyes and you guys are in the dark, but you know who you are. Thank you very much for sharing it with us. Your plant floor notes, that Tom trained the model on, so that's, yeah, really cool. So you know who you are. Thank you. [laughter]
26:49
Tom Hechtman: Yeah.
26:55
Mark French: Okay. So Tom, sentiment, what, what's the next step here? Could we combine sentiment with the fault prediction with the loss reasons? Like where do we go next?
27:07
Tom Hechtman: Absolutely. So you can combine different technologies together here. You can take that sentiment value and go with predictions or combine it in analysis and, yeah, absolutely.
27:20
Mark French: So can you walk us through what is happening behind the scenes of these ignition displays for, what's happening with the data in SepaIQ?
27:28
Tom Hechtman: Absolutely. So we're gonna take a look at the data flow here. Don't get misled by Ignition smaller. We just did it for the sake of explanation. Ignition's huge. Okay. [laughter], SepaIQ. We do have it in the middle. Everything on the left side is, data flow in it's producing data into SepaIQ. Everything on the right side are consumers going out. So on the left side we see Ignition there, we also see, spark plug, MQTT and all that. You can feed it up through Ignition, through MQTT if you want. But you could go direct spark plug, and then you see a database down there. So we've had this feature request many times over the years. Hey, we want to include other outside data into our analysis so we can actually read from other databases that were populated by some other system and include that in our analysis.
28:27
Tom Hechtman: Okay. So we collect our values from PLC manually entered ERP systems, whatever flows in through Ignition here, goes into our data groups and stored off in a time series format. So there's not a lot of joins or anything, it's just pretty straightforward. In fact, you open it up and you'll go, oh, I understand that. Pretty straightforward. So then later on, we wanna do analysis, or that could be, that could trigger the analysis to be recalculated, pull those values out. The streaming method, we can do, custom calculations, sentiment predictions on those values, those values all can be combined together into the analysis we're doing, grouping filtering, all that kind of thing into the results. So we have our analytics results and then those then go out, to be displayed on the screen. Okay, so our demo that we did that was the flow data changed re-triggered analysis, and it flowed out back to the screen, and it does it in a very performant way.
29:41
Mark French: So, Tom, quick question. A lot can be hidden in an arrow. What kind of broker do we have there between Ignition and SepaIQ?
29:48
Tom Hechtman: Well, we set it up so you don't need a broker in there. So it's a restful API, you could put a broker in there and use, either MQTT or Kafka. So, and we'll be adding other connectors in the future. And incidentally too, ignition, you saw event streams and you saw connectors in, connectors out and all that kind of stuff. Sources and handlers in that. And you see it here. Okay, so what's the difference, right? So this is really a data model intended to sit above ignition, and we, on the first diagram we have there to feed data up into higher level systems and to be able to go on multiple servers in a cluster do analysis. So you get very performant analysis. All right. Let's shift gears. Now we're gonna look at a Kafka example here. We have Ignition, everybody should be familiar with the designer. We got focus on the tags in the lower left corner there. And we're, we've got a UDT here, kept it really simple just for this to make it very clear in this presentation. We got a state and we have, like an equipment state, and then we have the state type.
31:03
Tom Hechtman: So we'll go over to the Kafka dashboard here, I think. There we go. Okay. And we have some topics here. We have test topics, and you see there's zero messages here. So this Kafka is a broker, and it just handles events coming in and it's gonna pass them out. So we will change, the downtime here in the machine state, which is put it into an unplanned type downtime state, and we'll record that off in which it then, was sent to SepaIQ, then did some analytics, and then sent a message up to Kafka. So we come in here, we look at it, we got a message, and we'll go in and take a look at that message. And we'll put that message into Notepad++, because this is not easy to read. A little easier to read when it's formatted. Okay. All right. This is not just, hey, value changed. This is like, wow, we did some analysis. This is our downtime for shift, and we are gonna update it in our, and there's our, clogged filler there, I think it was. Yeah.
32:31
Mark French: Feeder clogged.
32:32
Tom Hechtman: Feeder clogged. Yeah. So these results, this is not, in a UNS structure, this is kind of more a row structure. It could be column or structure, it could be nested JSON structure. It could be anything really. And then we'll go back to run mode here and we'll see another message show up over in the, Kafka dashboard there. And it's important to know that if we go back and modify data, that a message with the updated data would be sent out Kafka. If we're going into a database, so we have, customers that are going into like Redshift, and then they use I think Power PI to analyze outta Redshift and stuff, we'll go back and update the database rows with the correct data. So that's something that's just straight, Hey, collect your data, put it up higher. It doesn't typically do that. And we've had multiple customers ask us, Hey, on our MES data, how do we update it when we make changes? So the, this solves that problem. Okay.
33:45
Mark French: So Tom, for folks that might not be as familiar with Kafka, they haven't stepped through some of these processes. Can you walk us through the data flow that we just observed?
33:55
Tom Hechtman: Yeah, absolutely. So we start out the same way as we did before. In fact, it could be the same data. We could have that data collected and it could update the analysis and send to Kafka, but because this, we'll just talk through the Kafka there. So the values come in, and we collect them. That triggers an event to recalculate. Same thing. You can organize that data, go up, do custom calculations and sentiment and whatever else, key reason, downtime, detection, lot normalization, all those things. Then that all gets combined into a, you can do your grouping, like you saw in those results, all that kind of thing into contextualized data. And so now we've got a data record here. Now that can then go out to whatever, multiple, database off a, back to a, chart on the screen even, so, changing one value can trigger off multiple things and updates.
35:06
Mark French: Excellent. So Tom, who's using this today? Like, who's out there leveraging this?
35:12
Tom Hechtman: No one. [laughter] So it's early, right? Like sentiment, who has sentiment? All right. So, there are some companies out there that have similar type products, but we we're very focused on MES and manufacturing data and solving all those problems. But we're very excited to see what customers are gonna do with this and how they're going to capitalize on it. So.
35:39
Mark French: Absolutely. I think one of the most common things we hear about from the design side at Sepasoft from customers is they want to get more out of analysis. They want to push that pedal harder, they want to improve performance of their system. And I think this is, the tool to deliver that. And I think it's the architecture, moving those calculations off of Ignition, with the other enablement features, right? With contextualization, the custom calculation, the machine learning modeling as well, to really give them what they've been asking for. That's pretty exciting.
36:13
Tom Hechtman: Yeah. You said the key there. They've been asking for it. When we meet with customers and ask, how do I do this? This product is a result of that. And the fact that we needed to update our decade old analytics, so.
36:29
Mark French: Excellent. Okay, so we've gone through our demos. Let's talk about, this Sepasoft product roadmap. So I'll take the completed stuff. Tom can take the hard things. What's coming up in the future. [laughter] So what have we already done this year? What's been recently released? Well just this week, batch formulas has been released. That's great. What is batch formulas? Right? So, this is a feature set that compliments our existing batch procedure product. And, when you have a recipe for, your plant floor activity, whether that's kind of classic batch, whether that's workflow control for discreet, doesn't matter to us, right? You have various data items, right? Those parameters that are, important to that recipe. Well, now you can save off a set of those parameters with specific values. It's now versioned, you can execute off of that. And that really gives you the, out of the box capability to say, Hey, here's our process. But there's 15 different products that all have different settings, that need to use that process, right? So you can identify your formulas for each product, is really well contained. Tom, do you want expand on that at all?
37:47
Tom Hechtman: Yeah. What comes along with that is greater security also. So you can have your own formula or batch recipe states and then you can put security on those. So now you can lock out somebody from going in and editing a recipe unless they have the right credentials. You don't have to use that but that's required for regulated industries. We've validated this. We cannot modify it now so greater support for that came out with that release as well.
38:24
Mark French: In addition, one thing that many of you have asked us for is tracking user changes. So when a user does make a change to any configuration item, we want to know who made it, when they made it, etcetera. So that has been added. I call it the whodunit feature. You may not like that name.
38:43
Tom Hechtman: Wait a second, I came up with that. Whodunit.
38:51
Mark French: So the whodunit feature is out as well. Another feature set that we've released is the SAP listener functionality. Previously our calls to SAP from business connector and the interface for SAP ERP were always outbound initiated by Ignition. Now SAP can call into your Ignition instance and reference those remote function module calls and bappies etcetera that are supported. So SAP can can push data, can pull data, Ignition can talk to SAP and push and pull data as well so exciting options there for our SAP users. We've also continued to roll out additional perspective components. I believe the SPC sample entry components was in the release candidate that came out this week so that's exciting. Heretofore that was only in vision so yeah, I got a little clap on that. I'll take that little clap.
39:53
Mark French: And then we've also focused on general product stability. Had a nice sit down with a systems integrator who told us that they could see this over the past two years that they've seen a marked change didn't mean the pun, in the product stability from Sepasoft so really appreciate that and we're not done there of course. We have more work to do but that's been a development goal this year. Tom, you wanna tell us about what's next?
40:21
Tom Hechtman: Yeah. So golden batch comparison will come soon and this is you know I've got a real good reference over here in Fran that what is golden batch? Is it the best quality? Is it the fastest time? You know, what is it? What this is is an exception reporting. So you have your ideal batch, and then you compare that to any other batch. And it's like, okay, did take longer to do this? Did you execute this step more times? You know, you did a rework or something like that. You can have tolerances in there and select what you wanna show on the report and all that kind of stuff. So we call it kind of an exception golden batch. Which then brings up a good interesting question for the other part of that is, well, what if you combine SepaIQ with Batch and you start selecting some parameters to pass up into machine learning and doing that. Now you can do some pretty deep dive analytics so in comparisons between batches and stuff like that. So we're excited to explore that more and work with customers on creating that. So Q4 for the golden batch and then SepaIQ which you've seen, Q1 in 2025 is when that will be released.
41:42
Tom Hechtman: So we're going into beta very, very soon here, within days, I believe. So, and then in Ignition 8.3, we've got changes we have to do for that. You're gonna see the Gateway console get updated. That's fine. And then we have changed sets. So, you know, the way Ignition has human readable files of every resource in a project, and you can put them into Git. And you can have branches and you can do diffs and do all that kind of stuff. Well when it comes to MES configuration like material definitions and all that, that's in the database, right? And so we're gonna have that same type of functionality. You'll be able to go from the database to human readable files, check them into Git, do diff, all that kind of thing. So in addition to that... All right.
42:34
Tom Hechtman: Yeah. And I do have to say I'm sorry, I know it's a pain, right? In addition to that, it's a little bit like Git, where you'll be able to create a... Start a change set, make several changes. Oh, go away for a couple days, come back, make some more changes. It won't be on your live system until you commit it. You'll be able to export that change set, go to another system, import it, and we will see that on the enterprise syncing capability as well. So those change sets will synchronize across the... Between gateway servers. So that's coming along with Ignition 8.3. We don't want to have multiple versions stacked up. We just want the 8.1 version and then the 8.3 will have that. And then we're gonna take advantage of the event streams. So SepaIQ will tie into it and Business Connector will probably tie into it. So now when you get information from SAP, it will go through the event streams. Or you can push data out to SAP through the event streams or web services or what have you. So that's what you can look forward to on Ignition 8.3.
43:50
Mark French: Tom, when are we gonna have modules for 8.3? Everybody wants to know.
43:53
Tom Hechtman: When 8.3 comes out.
43:55
Mark French: All right. There you go. All right. Let's wrap it up.
44:06
Tom Hechtman: Yeah. So the two main points that I hope you walk out of here with are that SepaIQ, which we announced, is used to interface with higher level systems. So if you have an AI initiative in your company, many companies do, we're not trying to replace that or anything else. We're trying to complement that. We're trying to take that effort, which typically I've heard out there it's 85% of the effort, to get data in the correct context, to get it clean and push it up to that system. We're trying to simplify that and this is a tool to do that. Now, if you don't have an AI initiative, absolutely you can capitalize on the internal machine learning type capabilities. We're gonna be adding natural language processing, tie into open API or AI, open AI, local AI, those technologies. And we'll be a tool to those. So when you ask for production data verbally or typing it out or whatever, the results go back to that. So we're not stopping here.
45:19
Tom Hechtman: It's going to expand. And the other thing I hope you walk out of here with is that, hey, all the initiatives you have with AI, it's just as important to put them down on the factory floor. And that's what SepaIQ is all about as well. So the two main reasons it was created in the first place are those two main points. So I hope you enjoyed the program and you remember that as you walk out of here.
45:48
Mark French: One last thing, Tom, we don't have time to do Q&A, but I've got a question for you.
45:52
Tom Hechtman: Okay.
45:53
Mark French: Can you only train models locally? Can you only use locally trained models?
46:00
Tom Hechtman: No, no. So we have some models that are in there, but you can create your own models, you know, and you can select your features and your labels. Those are machine learning terms and train it. But you could train it on an outside system and then import that model into this system. In the future, we'll have so you can go out of web service up to another system and get the prediction and bring it included in your analytics. But those features aren't here today. What you saw today is what we have, so.
46:33
Mark French: Excellent. Thank you, Tom. So this QR code takes you to the SepaIQ landing page. That website is live. If you have more questions, please feel free to reach out to the Sepasoft sales team, contact information there and Tom will be on the manufacturing hub podcast which will be streamed live after the conference later today. So thank you very much for your time everybody and attention.
46:58
Tom Hechtman: Thank you. Oh, we do now. We do...
47:06
Mark French: So we actually... They added a few extra minutes for us so that we could get some questions in. So if you do wanna ask questions, we have some mic runners.
47:15
Tom Hechtman: Can we get Dan a mic?
47:17
Mark French: Yeah, let's get a mic down here.
47:21
Tom Hechtman: This is the biggest mistake of ICC that I'm just saying this but here. Can we get Dan a mic?
47:24
Mark French: Go on, come in here on there. Okay.
47:35
Audience Member 1: Hello.
47:38
Tom Hechtman: Hey, look at that.
47:39
Audience Member 1: So where's this sit in your stack? Does this replace MVS3? Is this a new module? Does this replace the analysis controllers? What is it?
47:50
Mark French: Yeah, great question. You want me to take that?
47:51
Tom Hechtman: Yeah, go ahead.
47:53
Mark French: Okay. Yeah. So this does not replace the control functionality of our MES suite on Ignition today, right? This is in addition to. So a design decision can be made. Do we want to continue using the existing analysis tools on Ignition or do we wanna do that analysis off Ignition on the SepaIQ server? Does that make sense?
48:20
Mark French: Sorry. That's a commercial question in addition to the technical question.
48:22
Tom Hechtman: Yeah, yeah. I can answer that. Yeah. So we recognize that with the customers. And if you're in good standing on your support contract, it's gonna be very favorable to you. So very, very favorable. Maybe even three verys.
48:45
Mark French: So yeah, some of that's TBD. Is there another question before we wrap?
48:48
Bryson Prince.: Yeah, if you have any, just keep your hands up. We have mic runners both up and down. They'll run to you, and then we can keep going from there, okay? But we've got one here.
48:56
Audience Member 2: The four-second training time was pretty impressive. But I wanted to know, have you tested on a real system to give us any accuracy numbers? Because four seconds is great, but if it's only 15% accurate, then it's nothing.
49:08
Tom Hechtman: Right, yeah. So two parts to that answer, I think. So the accuracy, the training accuracy, it tells you what it is when you train it. It'll also tell you if your feature... The impact a feature has, so one of the items you're training against. So you might find that that's not even a feature I should include, that it's not relevant, which is a big part of machine learning and determining all that too. But it will tell you the accuracy, but generally machine learning is not 100% anyway, so.
49:42
Mark French: Good question. Is there another question?
49:47
Tom Hechtman: Up top? Do we have one up top? Yeah.
49:48
Audience Member 3: Yeah, quick question about batch formulas. Is that specific to the batch procedure module or is that settings and changeover included?
50:00
Tom Hechtman: Batch procedure.
50:00
Audience Member 3: Okay. Thank you.
50:01
Mark French: Yep. Good question.
50:02
Tom Hechtman: You can think of it this way 'cause settings and changeover is settings, but there's no logic. Batch had logic, but no settings in a way. So it's putting settings on batch.
50:15
Bryson Prince.: Okay. Got a follow-up down here.
50:19
Audience Member 2: Also on the training, I wanted to know, Oh. Sorry. More on on the the training setup, do you... Like what percentage of your training set, validation set, test set are we looking at? Is that configurable? Is that something we can play with and also what sort of models are we allowed to mess around with? Are we using ANN's or random forests, stuff like that?
50:45
Tom Hechtman: Yeah, so it's XGBoost, and we'll probably add others later on. So you have various options in XGBoost, so you can play with that. And you get to configure the parameters for the training. So you can specify, you know, the various parameters included in that training and your test set percentage as well. We have any more questions before we wrap up? All right. Do you have any final statements?
51:22
Mark French: Yeah. Great questions guys. Thanks for the time and of course, you know find us at the booth Manufacturing Hub podcast later today with Tom and if you're keen on learning more about batch procedure and formulas, Patrick Lee will be leading a workshop during the virtual ICC workshop. So sign up if it's not already sold out. So thanks everybody.
51:43
Bryson Prince.: Thank you Mark and Tom.
51:43
Tom Hechtman: Alright. Thank you.
Want to stay up-to-date with us?
Sign up for our weekly News Feed.