Turning Enterprise Data Into Decisions More Quickly
How to Leverage Ignition for DataOps
60 min video / 47 minute read Download PDFSpeakers
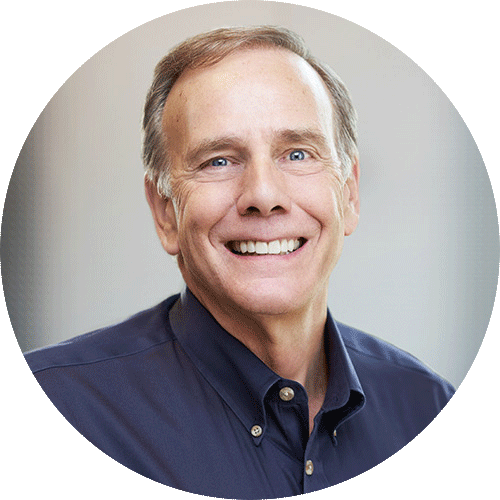
Don Pearson
Chief Strategy Officer
Inductive Automation
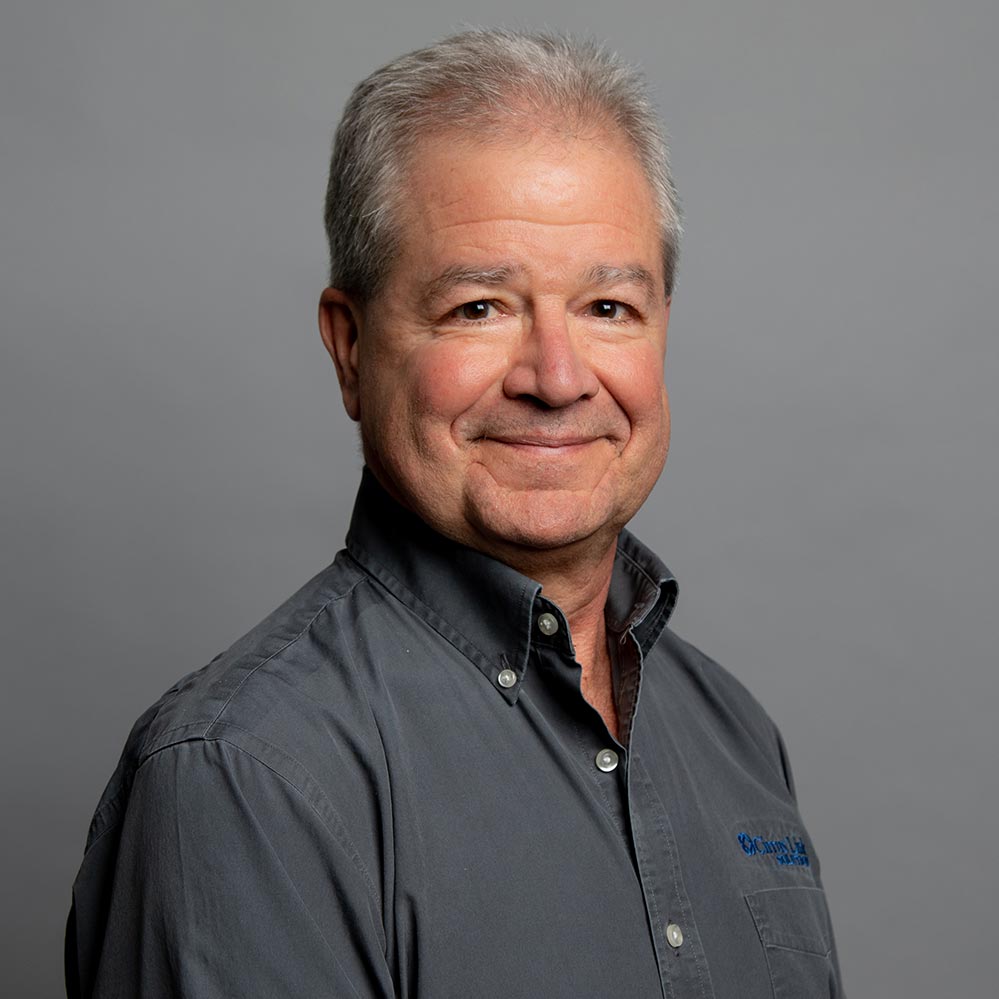
Arlen Nipper
President and CTO
Cirrus Link Solutions
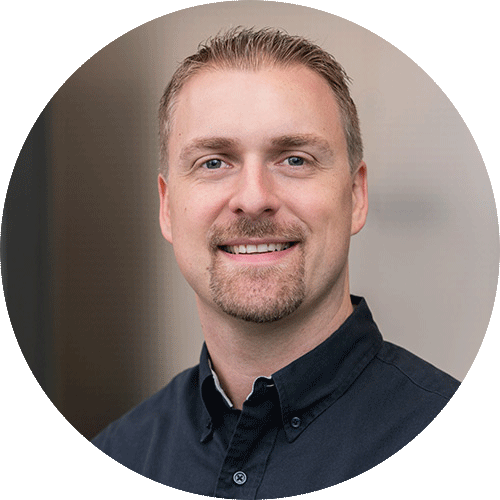
Travis Cox
Co-Director of Sales Engineering
Inductive Automation
Is inefficient data management standing in the way of your company’s IIoT or Digital Transformation objectives? As companies struggle with making their data more useful, many are adopting a methodology known as DataOps. The good news is that DataOps can help you collect, deliver, and leverage data faster and with greater accuracy — and with Ignition’s unlimited industrial application platform and its support of the powerful MQTT protocol, implementation could be easier than you think.
In this webinar, join experts from Inductive Automation and Cirrus Link Solutions to learn more about what DataOps is, why it’s important, and how industrial organizations can start putting it into practice.
- Learn about getting your data into a usable form
- Get insights for better speed and quality of data analytics
- Learn the requirements of a successful enterprise solution
- Ask your questions about DataOps, IIoT, and more
Co-presented by:
Webinar Transcript
00:00
Don Pearson: Welcome everyone to our webinar today,"Turning Enterprise Data Into Decisions More Quickly: How to Leverage Ignition for DataOps." My name is Don Pearson, I'll serve as the moderator for today's webinar. If we're to look at today's agenda, I'll be introducing Ignition and Cirrus Link Solutions, and today's speakers. We talk about what DataOps is and why it's important and how Ignition can be used for DataOps. We'll give you a short demo in Ignition just to glance at Ignition by Inductive Automation, it's an universal industrial application platform for HMI, SCADA, MES, and IIoT. Actually, Ignition turned 10-years-old. Last year, it's used by 54% of Fortune 100 companies and over 35% of Fortune 500. A couple of bullet points on the screen here that differentiated it. It's got an unlimited licensing model, cross-platform compatibility, IT Standard technologies, scalable server-client architecture, web-based, web-managed, web-deploy designer in clients, modular convertibility and of course, rapid development and deployment tools. In the software stack you can see that basically, this is the modular nature of Ignition. We have a full slate of powerful Ignition Core Modules and third-party modules.
01:21
DP: This allows you to develop customized industrial applications that really fit your unique processes instead of the other way around. As you can see here also, Cirrus Link is one of only two Inductive Automation strategic partners, in the upper right there, under the IIoT modules area. Strategic partner Cirrus Link in that capacity, develops and supports the IIoT, cloud, and EFM modules for Ignition. They bring 30-plus years of SCADA and Telemetry experience to the table. They provide innovative leadership in the industrial space for IIoT and digital transformation, and they've been really integral in the creation and continued growth of the MQTT protocol, which we'll discuss a little bit further today. They developed a Sparkplug's classification and contributed to the Eclipse Foundation, and is now part of the Eclipse Tahu project. And all of this is really why they're trusted by Amazon Web Services, Phillips 66, Tyson, Georgia Pacific and a whole host of other enterprises. Our guest speaker is the President and CTO of Cirrus Link Solutions, he is also the co-inventor of MQTT on the network. I'm also joined by our own co-Director of Sales Engineering, as most of you know, Travis Cox. So, Arlen, let me just start with you. Please, I gave you a brief introduction, but take a moment, tell us more about your background and your role at your company.
02:55
Arlen Nipper: Well, thank you, Don. We're looking forward to this webinar today. My background, I guess is, I've been doing industrial communications in one form or another for over 40 years. Cirrus Link was actually formed for us to better leverage MQTT in what we knew, since MQTT was invented for mission-critical real-time SCADA systems, we knew it was applicable to this new emerging IIoT, and that was the focus of starting the company, and now in working with Inductive Automation, we're able to actually bring that to fruition.
03:37
DP: Thanks, Arlen. Travis, even though I said, probably most people know you, please take a couple of minutes. Introduce yourself a bit better.
03:45
Travis Cox: Yeah. Thank you, Don. My role here as a solution architect, in our team, we have been helping customers with this subject for quite some time now, in terms of being able to get access to more data, to move that data around, to integrate with other systems, whether it's on-premise or whether integrating with the cloud. And it's something that the Ignition platform has really been able to do from the very beginning. It's been very important for Ignition to be able to do more, get access to more data, and do more with that, and so I'm really happy to be here and chat about my experience.
04:22
DP: Thanks a lot, Travis. Thank you also, Arlen for being here. I know also that a lot of you were joining us from the ARC Industry forum last week. ARCs theme this year was, "Accelerating Digital Transformation in a Post-COVID-19 World." That's exactly what a lot of companies are trying to do. They're trying to accelerate their digital transformation and keep up with the pace of technological and business changes, but they're being stymied by a whole slew of data problems. There are, as many of you already know, inefficiencies in trying to access, compare and integrate data and in making data available. There's inconsistencies with the data, which is coming from a variety of different sources, and result in lack of data context, and often data might be accessible, but it's not in usable form. Sort of, as you have said many times Arlen, you sort of move stuff up to the cloud, but you're really just kicking the can down the road a little bit 'cause you don't really get it in a usable form. So, we really don't want data just for its own sake, we want data to help us make better business decisions for our organizations. Arlen, can you just elaborate some more context around this point, the kinds of data problems and why they matter?
05:45
AN: Sure Don. Well, if you look at our history, I mean, just look at the OT, SCADA, HMI sector. We're coming off of 40 years of having a paradigm. And that paradigm was: We have a register and a value, no matter what the poll response protocol, we have a register and a value. So for example, we have Modbus register 40,100 has a value of 200. We don't know if that's 200 gallons, 200 degrees. We have no idea. So what we do, we double click on that tag and we give it context. We give it engineering units, we may scale it, we may give it engineering-high, engineering-low. But now with digital transformation, we have to do that if another consumer gets that Modbus register. We do it again and again and again. So what we're talking about here in this digital transformation is, our job is to provide tools to make data humanly understandable to all the other enterprise applications that need to consume it, and that's gonna be really the focus of this session.
06:57
DP: Thanks, Arlen. I really think that puts a better framing around what the scope of the problem is and really what the challenges are for organizations. Fortunately, there is a solution to these problems, which more and more companies are choosing to adopt and it's called DataOps. DataOps is, by definition, an automated process-oriented methodology, which is used by analytics and data teams to improve both the quality and reduce the cycle time of data analytics. So DataOps addresses the data architecture needs of industrial companies as they adopt Industry 4.0, digital transformation and smart manufacturing. It seeks to really improve communication between data stakeholders, and to really align the way that a company manages their data with the goals that they have for that data. This general process is usually called DataOps, but it is sometimes called data harmonization or data cleansing. DataOps has some similarities to DevOps. Both of them combine people, processes and tools to deliver applications or data more efficiently and both encourage organizations to break down internal silos so that the end product can be delivered more quickly and with higher quality.
08:18
DP: And it's important to remember that DataOps, like DevOps, is a methodology rather than a product or a solution for a specific position or a specific team within the organization. It can only work if it is shared and adopted widely within the organization. So let me turn to you, Arlen, and you, Travis. Before we move on to talking about implementing DataOps, do you have any thoughts you may have about the definition or purpose of this methodology? Maybe I'll start with you, Travis, and then over to you Arlen.
08:52
TC: Yeah, so we could argue that we've been doing DataOps for quite some time. A lot of customers have been doing that. And generally speaking, they're doing that within a siloed environment, within a SCADA Solution in particular. And so it works really well to basically take the registers Arlen was saying and add the context to it and be able to put it on screen and work with it. But today the world of digital transformation and the world of wanting to do more with that data, give that data to business, that methodology stops and starts at SCADA and we need to be able to expand it. The idea of DataOps is to, again, I'll emphasize the widely adopting the idea of this, of being able to get that data and bring it into an infrastructure and leverage it a lot more. But in order to do that, we have to leverage modern technology and standards in order to get there, which we'll be going into a lot more detail here.
09:52
DP: Great. Thanks, Travis. Your thoughts, Arlen.
09:55
AN: Well again, the same thing is, the forest for the trees, Don, is that we've had this capability within Ignition, we've had this capability, from what Travis said, the very beginning, but now we're thinking about it differently. One good example I always think of is, where we look at data from EFM devices, electronic flow measurement, and we'll bring those tags in, and from an operational standpoint, we're quite happy with all of these crazy enumerations that we have in our operational systems. "Oh a zero means this, a five means this", but we know that. We have that tribal knowledge within our OT group. But now our job is to get that out and get it out to other consumers of our information. So with Ignition, with things like UDTs, now we can take those enumerations and create string tags within our UDTs that further defines that. So that down the line, instead of a zero, you may get a value that says "Office material is stainless steel", or you may get any of those enumerations that we deal with today, operationally. We need to think about making those easier for everybody else in the enterprise to understand, and I think to a large part, that is the definition of DataOps.
11:19
TC: Yeah and I'll add one more thing to that, Arlen, which you say a lot of the time, you said it here. We'll emphasize it yet again, is that we want to be able to do all of that work at once and leverage that work everywhere else. If we define that information, we need to be able to discover that and those objects everywhere else, and that's a really important thing. It can't be siloed. It has to be brought to the entire infrastructure.
11:43
DP: That's a great point, Travis, and I think it really emphasizes that, as we bring OT and IT organizations together, you really basically have to have a nomenclature, and naming, and conventions, and modeling, and asset occasions where basically, everybody can understand and be on the same page, if at the end of the day we're gonna get better decision-making. So, let's just talk about implementing DataOps. How you do that actually depends a lot on the nature of your organization and what you want to accomplish, but here are some just general guidelines for implementation, which we believe apply across the board. You need to actually first democratize the data. In other words, to make data available across the enterprise with as few restrictions as possible. You should leverage platforms and open-source tools as much as possible. You should build a model for your data to organize and standardize data elements, and you need to facilitate cooperation between departments because no one department or team can make DataOps happen. For most companies, these steps represent a big shift, a huge paradigm shift in the way they currently think and actually how they do things.
12:58
DP: So while technology solutions aren't, if you will, the magic bullet for DataOps, they are an important piece of the puzzle. I guess I'm mixing my metaphors here, I apologize for that, but they're a big piece of the puzzle. Specifically, DataOps solutions can perform data contextualization, standardization and provide secure data flow to the various consuming applications that are running at the edge, whether on-premise data centers or in the cloud. We have to emphasize that in order to implement a DataOps solution successfully, you need to have the right foundation. Ignition actually meets all of those foundational requirements. I think I need to get into a little bit more detail, and I think perhaps this is a perfect time for me to hand it over to you to dig into that, so Travis, why don't you take it from here?
13:50
TC: Perfect, yeah, so if you rewind and go back to the roots of Inductive Automation and Ignition, our founder, he was frustrated with the software on the market at the time, because of proprietary natures, because of being siloed, because of licensing restrictions. And ultimately we were set out to alleviate all of those, to break down those barriers and to provide a solution that we can grow with, that is a foundation for us to build more, and that is really why Ignition fundamentally is perfect for DataOps. And we can go into some of the specific points. First and foremost, Ignition is built on open standard technologies and tools. We, again, didn't wanna think proprietary. We wanna leverage standard SQL databases, leverage standards like OPC UA, and MQTT, which we'll see more here today. Be able to integrate with other solutions quite easily through APIs or other direct integrations. And Ignition never owned the data, it might connect and be the source of driving that data somewhere else and letting you take advantage of it, but it never locked in to a location there. And Ignition also has an SDK, it's a foundation, not only are there functions that it provides out of the box, but then it's possible to extend that and do more with that data, and with the system there.
15:18
TC: Ignition is also highly scalable. We can use it anywhere from a local data collection node or an HMI, all the way up to an enterprise or a large complex system that has many different servers there. And we also look at this now, a distribution with Edge being a big part of this, having the Edge play into a centralized solution. Ignition is really price competitive, this is probably one of the biggest benefits of Ignition. From the beginning, not only do we want the functionality to be open to leverage new technologies, but wanted the licensing model to be unlimited so that you can get a single license that is unlimited in use, in terms of tags, screens, clients, connections and more. And that really is an important piece in order to do DataOps properly. A lot of times systems, a lot of data stranded in the field because of license restrictions. You had to cut corners or you had to cut what they wanted to bring in because of that. Ignition opens that door, allows you to connect to more devices, get more tags in the system and do more with that data, as you are gonna see here today. That's also OS agnostic, so you can run Ignition on any flavor of Windows, Linux or MAC, and that's important, because we can leverage any kind of existing hardware.
16:42
TC: We can deploy Ignition literally to anything, whether it's physical hardware, virtual machines, containers, managed services, it can go anywhere. And having that allows you to do some pretty interesting things. Especially at the edge of the network, we can run Ignition in a file computing environment or on existing managed switches or embedded PCs or constrained machines that are out there, and that makes it a lot easier to manage going overall. And so it just gives you that flexibility to do more with it, and I think some of the important things here is not only is it OS agnostic, but it's really easy to install, to manage and configure. So we can get up and running in a couple of minutes, we can fully configure your system and continue to add on to that as we go forward. And we provide all the data connectivity, so there's drivers to different Legacy devices or various polling protocols built into SQL databases to OPC UA, MQTT, web services and a lot more. And because of that, that allows Ignition to do more in terms of DataOps. And I think fundamentally, Ignition is really rooted in OT. It's OT-driven and proven, but it bridges that gap between OT and IT because it speaks the languages that IT understands and it follows the technology and various paradigms that IT has, especially today and around security and more.
18:17
TC: It's not looked at as a system that we can't support, it's looked at as, "Now let's bring the managers of OT and IT together and put in a solution that's gonna work, holistically, across the board." And that's, I think, the biggest driver here, that's a people change, it's getting commitment to the idea of wanting to do more with that data and really fundamentally solving it from OT up rather than from IT down. Now, there are a lot of other important reasons why Ignition is a strong foundation for DataOps and for that, we're gonna turn over to Arlen, so he can not only talk about that, but show us how some of this can actually work as well, so Arlen over to you.
19:01
AN: Right, the way we look at, again, we were talking about the force for the trees, and the fact we've got all of this capability with the Ignition platform, so let's kind of dive into that a bit more. So from a DataOps standpoint, Ignition gives me the ability to connect to my OT data, like Travis said, I've got my protocols, I've got my OPC UA client capability, I can go into databases and get data. So that lets me start pulling in all of this brownfield tags from all of these different sensors and devices in the field and start providing that context that we need. So first of all, we know that within Ignition, we've got the ability to take a process variable, a measurement, whatever you wanna call it, and we can get it into a proper format, so we can give that Modbus register, engineering units and ranges and give it a proper tag name. Now, the other thing we're gonna start leveraging on here is the ability and the tools to create data models at the edge. So, there is this tool called UDTs, User-Defined Data Types, that lets us take those tags and start grouping them in a model. And now that model, if we could take that as a single source of truth, now we can scale that within the enterprise.
20:20
AN: So imagine being able to create a model, and instantiating that, creating an asset, and then that asset has the measurements that we need, the scale in engineering units. That notion of a measurement object, that also contains the timestamp, contains the data type, so that unlike register value pairs, once they've gone away, they become kind of ephemeral. How do you put them back together? Where with UDTs and Ignition objects, I can go back a year, two years, three years from now, find that single source of truth, and have all of the metrics that I need for that process variable that I measure. It gives us the ability, then from the edge, to do simple connect and learn instantly, and we've eliminated the requirement to be editing these tags upstream. Now, really, what we're talking about here is that the single, I always say this, wherever possible, we should be using tools on platforms, not coding on our operating system. And we'll go back to discuss a lot of the projects that we've been involved with, where we do have customers trying to get their information in the cloud solutions.
21:40
AN: All of you know Amazon, Microsoft, Google, IBM, all are starting to provide secure containers that went on the edge, and that's great. But those secure containers will let you write code, and what we're finding is that if you're writing code to get these process variables and to get them processed to get them in the cloud applications, that doesn't scale very quickly. But, if I have tools, where one engineer works on and then he can hand it to the next engineer and those tools are consistent, then we can leverage the tools on Ignition to create our DataOps capability. Using MQTT, now, we can get that notion, that mantra we've been talking about now for five years, is that, "In the modern world, we should think wherever possible about connecting our devices to infrastructure, and not to applications. If we think about what we've been doing for the last 40 years, we take a perfectly good piece of equipment with a protocol, we have a Polling table, we tie that protocol to an application that's going to poll that device and get that information. Now, we all have good intensity, "Hey, in the future we'll change that Polling table. In the future, we'll get some of the other stranded data.
23:02
AN: But typically, we all know that's hard, if not impossible to scale. Whereas if we look at leveraging MQTT, publish subscribe infrastructure, that lets us take devices, that can publish information serendipitously, and then other applications can subscribe to that information or not. So, we get this structure where, now we've this ability for a device out in the field to come up, publish all of the information that it knows about, and then have other applications subscribe to that resulting in a one to many architecture, which DataOps digital transformation is going to require moving forward. With the MQTT modules that Cirrus Link have developed for the Ignition platform. Ignition is an MQTT Sparkplug enabled platform. Now, Sparkplug is an open source standard that was created by Cirrus Link, but is now managed by the Eclipse Software Foundation. So, it's very important to understand that we've taken this Sparkplug speck and given it to an organization that is software centric, and that from that organization, we have the Sparkplug steering committee, and the sparkplug specification can be downloaded directly from the Eclipse Software foundation.
24:28
AN: Sparkplug helps MQTT clients connect seamlessly and integrate data between your sensors, devices and gateways within that MQTT infrastructure. Because of the Eclipse Foundation and many of the organizations that have joined, Sparkplug is rapidly becoming the standard for IIoT connectivity for cross industry interoperability and digital transformation. Everyday, I'm always amazed, even yesterday, with a very large equipment manufacturer, to discover that they are developing MQTT Sparkplug for all their equipment for this very reason. Now, very quickly, what does Sparkplug do for us? So, the beautiful thing about MQTT is that you can publish anything you want on any topic. So, if you look out in the world today, look at Facebook Messenger, that uses MQTT, look at all the cloud service providers, they use MQTT as their ingest, as well as typically they use MQTT for all of their inner process publish and subscribe infrastructure. OEMs were starting to implement MQTT. Four years ago, when we looked around the ecosystem, a lot of people were doing MQTT, were talking about MQTT, but the problem was there was no interoperability. Everybody had a different topic namespace, and everybody had a different way to represent the payload, typically, where your process variables and your measurement information resides.
26:06
AN: So, Sparkplug simply defines an OT-centric namespace that we can all agree on. Now, what does that do? That gives us that auto-discovery plug-and-play capability, because now we know what to go subscribe for. Now, once we subscribe to that we've created an OT-centered data model and asset structure. That means with MQTT Sparkplug we can publish a model from as far out at the edge of the network as possible, then that model can be consumed by all of our other applications, to the very intent of DataOps. Now, applications can discover models that better describe the information that those devices in the field are providing to us. Then we've defined, again, an OT-centric and extensible process variable payload, again, creating that measurement object that contains everything that we need from a historical standpoint. It's got the time standard, milliseconds, it's got the engineering units, the ranges, the data type, the current value, and any other property that you wanna add to that measurement. Now, the other thing that Sparkplug did was, it better defined what was meant in the state and management capabilities of MQTT, because if we're really doing an operational system, even from a command to control standpoint we wanna know the state of all of the MQTT clients within the infrastructure in real time.
27:45
AN: And if those devices would fall off, or if our network gets interrupted, we need to know in real time that those devices are no longer connected, and be able to take appropriate action when those connections drop. So ultimately, what Sparkplug does for us, with MQTT, is that it establishes a single source of truth for models, assets, and tags at the origin. Now, let's put this in context. Todd Anslinger is one of the executives for IIoT for Chevron, and he put together a few slides here that says, "Well, all this technology is great, but what problem are we actually trying to solve?" From a Chevron standpoint their job is to take care of facilities that produce natural gas and oil, and those facilities they were commissioning approximately every week of the year. They've gotta integrate those into their process control network, and then, from there, integration into their enterprise business systems. On top of their own facilities, on an ongoing basis, Chevron has property swaps and purchases from other oil companies, and again, they've gotta go in and make that system look like what they want it to look like, I.e., a DataOps problem. The challenges were even though we're doubling, tripling, quadrupling the number of property swaps, and the number of facilities, oh, guess what? Manpower is only gonna go up by 30%.
29:24
AN: So if you do the math we've gotta reduce the time it takes to integrate and create those DataOps definitions. There are five things that Chevron wanted to measure here from the standpoint of integrating a PLC, the local touchscreen to that PLC, the control room graphics, getting that information to a PI historian, and into the decision support center.
29:52
DP: Arlen?
29:52
AN: And our integration to that point... Yeah.
29:54
DP: We had a question that I think you might wanna answer now quickly. Someone says, "Can you better define assets in this context?" I'm only interrupting, 'cause I think you might wanna better define assets in this context and then continue on.
30:06
AN: Oh, sure, Don. Great question. An asset could be a remote tank farm, a booster station, an oil well, or an oilfield, so consisting of could be EFM devices, electronic flow measurement devices, PLCs, and sensors across anything that Chevron does. That could be in a pipeline control system, or it could be upstream, like in a natural gas gathering system. And then those things that we're talking about, as in this graphic, those would be valves, those would be tank levels, vessels, state of vessels, pumps, anything to do with an oilfield pipeline control system, that would be an asset. And now I've gotta take that asset and all those devices and I gotta forklift it over into my Chevron infrastructure. So what Chevron did was they kinda took the Sigma Six approach to this. And let's compare the time that it takes to propagate a standard object addition through the system. Test number A, or number one, is we have a vendor PLC, with the same touchscreen, but different HMI and integration to OSI PI. The second test was a single vendor. It should be easier, the same vendor for the PLC, the touchscreen, the HMI, and the historian, and then thirdly is let's look at this from a pub/sub MQTT standpoint.
31:40
AN: So, going through that with the measurements that they took, you can see here, and so with an integration with the PLC, the touchscreen vendor and HMI were different, and the test was at a single Tank object. In other words, my job is, we're gonna add a new Tank from this facility, from this asset, and we need to get that Tank integrated into our infrastructure. With different vendors, it took 22 minutes to take that single Tank and integrate into their infrastructure. With a single Vertical Vendor solution, it took 13 minutes. But the Publish and Subscribe system using MQTT and Sparkplug, it took them 4.5 minutes to integrate that new data point into their infrastructure. Let's take a look at how we leverage all this. This is an architecture of the demo that I'll be doing here, and we're gonna cover a couple of integration points, if you will. One is, we're gonna start really simple, and one of the things I wanna point out to everybody on this webinar is that we've got the capability of download-it-and-do-it, so I'm gonna show you a very simple implementation using Ignition Edge, running on a Raspberry Pi, integrating Modbus PLCs, representing a wind farm and getting all that information into, not only my Ignition system, but into AWS IoT SiteWise.
33:17
AN: That's something that everyone on this call could do this afternoon. To Travis and Don's point, download Ignition, install it, configure it, like I'm going to show you, and you could have this up-and-running this afternoon. Then we're gonna go and look... We've had a lot of interest in LoRaWAN sensors that are available now, and trying to integrate those in. Especially in terms of things like machine vibration, Tank levels, temperatures, and how can we integrate this kind of mishmash of all of this LoRa technology that's coming out, apply a DataOps mentality to that, and be able to integrate those sensors very, very quickly? And then finally, let's look at a topology where we might have an Ignition system already existing in a factory at their facility, and now with the newly-introduced BACnet IP capabilities that is native to Ignition, maybe we could look at integrating-in part of the building management system, that's already in a factory, that's already in the facility, along with integrating the manufacturing lines. The first thing we'll do is we'll start with a very simple Ignition Edge. What I've done here is, this is the Ignition Designer running-on on a Raspberry Pi type device, and we wanna take and create this notion of a wind farm.
34:46
AN: The first thing we're gonna do is leverage this capability in Ignition to build a UDT. And in that UDT, I said, I wanna build a wind-turbine model and that's gonna have some parameters, I might want a location and who the supervisor was at that location. And then of course, I want my measurements. I want my RPM, my torque, my wind direction, my wind speed. Now, in this example, we're coming from a mod-less PLC, but tomorrow, this could be an Allen-Bradley PLC, and the day after that, it could be a Siemens PLC, and the day after that, maybe it's a BACnet IP device, but we've built the model now that we can standardize on. So now that we've got that defined, let's go and see how difficult it would be to get that into our infrastructure, and again, in this case, we just wanna go to AWS SiteWise. We'll look here in our SiteWise service and it lets us build a model, it lets us substantiate that to build an asset, and that asset is gonna have measurements. Now, if we look here, we can see that we don't have any models and we don't have any assets. So, what we're going to do, let's go back to our topology drawing.
36:03
AN: We're going to take the MQTT Sparkplug and we're gonna connect to the MQTT server in Amazon, AWS IoT core, and we're gonna use a service that we've got called Sparkplug SiteWise Bridge that's going to build those models up automatically into our SiteWise Service running here. What we're gonna do is, we're gonna go into the dashboard for this device, and we can see here, we've got all these modules running on the Edge. We've got MQTT transmission. How hard is that? Well, the first thing we're gonna do is look at our servers, and I've got this AWS IoT Server defined, and well, I've gotta give it the URL that I got when I set up my Amazon service, and I'm gonna provide the three TLS "certs" that I've gotta have to connect securely into AWS IoT. Now, once I've got that set up, I simply come in and enable my MQTT connection. At this point, what has happened is we have this Edge device, representing wind-turbines, that's publishing Sparkplug information into AWS. Now we go back to our IoT SiteWise. One minute ago, we didn't know about any models, but now we have a wind-turbine. And look at the wind-turbine model and see here... Here's our attributes, our location, and our supervisor. And here are measurement definitions, we've got a RPM, a wind direction and degrees, a torque, wind speed and our OverDrive status.
37:57
AN: Now that we've built the model, we know we had two wind-turbines in our Edge, so we're gonna look at our assets and see that we have our two wind-turbine, turbine One and turbine Two. And if we look at that, we can say, here the supervisor is Arlen Nipper, and the location is we're in Kansas City, and here's all of our real-time measurements of dating into the time series database and site-wise automatically. So there is a very simple example of how we took Ignition Edge, built a model and populated that directly into a service in Amazon. Now, if we pop out here to what we can do with LoRaWAN, if any of you are familiar with all of the LoRaWAN devices that are out there right now. We look here and we want to take advantage of this new Yokogawa vibration sensor or a radio bridge level sensor, but before we do that, again, let's jump into this notion of, let's define a data model first, so if I go in and look at a Sushi vibration sensor, I wanna make it humanly understandable, so I've taken all of the binary data and I've created a health report, I've created my LoRa metrics for that particular device, of course, the measurements, and we've provided all of those diagnostics that nobody ever used because they can't figure out how to break those out.
39:39
AN: So now we've got the ability on a level transmitter, a vibration transmitter of 4-20 milliamp sensor, and we wanna connect that in because at our factory, it might be very valuable to go into the factory and wirelessly be able to start monitoring vibration. So what we're gonna do is look at our Ignition Gateway now, and this is our gateway running on EC2 instance in Amazon, and we're gonna go in and look at our designer very quickly, and here's our Ignition Edge, but notice that we don't have anything connected, we're not connected into that MQTT infrastructure, so we're gonna connect to it by going into MQTT engine and enabling it. Now, what that Ignition Gateway has done now, is going out to the MQTT infrastructure, and it's looking at those devices that we've got coming from that gateway, that's got the LoRaWAN sensors on it, and now we've got these devices, here's our Sushi sensor, and here's all of the measurements from that sensor. So, we published a model from the very edge of representing a very complex vibration sensor, and now our plant can start using that information.
41:07
AN: So finally, let's take a look at the Ignition Gateway itself. And again, in a DataOps model is that I've got all of these tags coming in, and you people using Ignition app, probably you are doing this today, and we're gonna get out of this notion of a smart building tag provider, and in that smart building, you're using BACnet IP or Modbus, I might be able to connect to the cooler and to the compressor, and that those are parts of the chiller, and maybe I wanna put that in a facility, so now what we've created here is a hierarchical UDT, where I've got the notion of the facility that has a chiller, and that chiller comprises of a compressor and all of its measurements, and a cooler, and all of its measurements. So now that I've defined that UDT, come back to our tags and we have the notion of the smart building, a campus, a Line one area where we wanna monitor some of our environmental conditions and our chiller, compressor and a cooler. Now, I have that information again, I wanna get that conveyed to my cloud services as an object, and this one happens to be a hierarchical object, so we'll go back and right here, now we're gonna plug this Ignition Gateway into that same MQTT infrastructure on Amazon.
42:37
AN: So here we've got our same MQTT transmission pointing at the same MQTT server, this AWS IoT, and we're gonna go here and just make sure that our smart building transmitter here is enabled, and it is, we're gonna back to general and we're gonna plug into the infrastructure. Now again from a DataOps standpoint, I'll just point out while the models are being built, that typically we all think of this as asset frameworks and we want to know asset IDs and models and locations and things like that. Well, now we're able to build that, so here is my chiller, and here's my facility, and notice that in my facility, I've got my attributes, my location and my supervisor and I've got some measurements from it, maybe that facility gives me current wind speed, wind direction, current temperature, and again, I'll go back to our actual assets that we've built out, and now I've got my smart building with the chiller and the compressor, so I literally five minutes ago, we didn't know anything about wind turbines, we didn't know anything about LoRaWAN sensors, we didn't know anything about maybe our building management system, and now not only do we have that integrated into Ignition, we've got it seamlessly without any coding, all by configuration and tools, have it all the way up in our cloud services as a single source of truth. So with that, Don and Travis, I'll turn it back to you.
44:25
DP: Arlen, thanks. That was good. Maybe just to wrap up with you and with Travis, I think there's a lot of insight that comes from that kind of a presentation, but let's maybe summarize the discussion. So Travis, you watched the demo, you've been involved in this for a long time, share your final overall thoughts and then I'll give you a wrap-up opportunity too, Arlen. Travis?
44:48
TC: Yeah, so I think the demo, it really speaks for itself in terms of the power that's there. I think it's really important as we look at our organization and look across different facilities or different sites, and really fundamentally try to solve this OT challenge. Get that mapping done once, build those objects, try to standardize across multiple locations. And leveraging this technology, we can bring it up to higher layers and if you want to leverage the power of the Cloud to do things like analytics or machine learning and go up further, these services like SiteWise and others could really help you to achieve that. But if we can have the models that we build on-premise automatically discovered in the Cloud and consistent across the board, it allows us to really go a lot further with all that data, and it's not a hard thing to solve. We're talking about if you already have an existing system in place, we can build some new objects and new models of the existing data that we can bring up. Fundamentally, an important thing needs to happen, a shift change that people have to accept, but there's a lot of power that it can bring to the table.
46:11
DP: Thanks, Travis. Arlen, your wrap-up thoughts?
46:14
AN: Well, again, Don, to a large extent, it was forest for the trees in the standpoint of when we first started doing Sparkplug and we had this notion of a process variable, single source of truth, and then we started throwing those process variables up in the data lakes and then we saw people struggling with, "Oh, well, gosh, they're putting it back together the same way we had it when we had it down on the UDT to begin with." And that was kind of that epiphany of going, "Wait a minute, we've got the ability to publish UDTs. Let's go ahead and publish a model. Let's start trying to make that data humanly understandable by everybody else other than, again, those operational people that have that tribal knowledge." So to me, it's been very exciting to be able to go from the Edge with tools. Now notice, I just wanna point out, in this demo, I didn't write a single line of code. Everything was done with configuration and everything was done with tools that can be extendable and now I can scale. Whereas if I was writing Python code in the container and then having to say, "Well, gee, Mr. Operational Guy, every time you change from another plant, you're gonna have to go in and I need you to edit these five lines of Python code." That's not gonna scale and that's what we were seeing and I think that's why this is really taking off.
47:39
DP: No, that's a very good point. Just for those who are new, we told you a lot about Ignition today. Really invite you to just try it, go to our website, download it, full version. Takes about two, three minutes. You can try the modules that were talked about, Perspective, Vision, SQL Bridge, Cirrus Link modules. So it's a quick three minute download, please take advantage of that. Secondly, every year at ICC we have a Discover Gallery, and so if you've built an exceptional Ignition project, you'd like the rest of the world to see it. I invite you to submit to the 2021 Discover Gallery. It's a video showcase of our top Ignition projects from around the globe and it's part of our virtual ICC Conference, which will be in September this year. So you can submit your project at our ICC conference website. The deadline is April 30th, so go ahead and get to it. Additionally in one week, we've got another online event that you don't wanna miss, it's called Ignition Community Live: Ask the Engineer, and it features our other co-directors Travis Cox and Kevin McClusky. So if you have a chance there to ask any questions you want about SCADA, IIoT digital transformation, machine learning, whatever, and there will be a registration link in the webinar recording email. You'll receive it tomorrow.
48:58
DP: So join us on February 25th, it will be a lot of fun. Kevin loves to be challenged. And with that, if you want a personalized demo of Ignition, there's a number here. We have our account executives here, be happy to do that for you. So I think we're at the stage where we wanna get to Q&A, so one of them was basically a question related to connection to other systems. "So can you please share more insight in the easy integration with ERP, SAP, JDE, Oracle Manufacturing, etcetera." So Arlen or Travis take your shot at that.
49:40
TC: What we showed here today was a lot about taking data from PLCs or devices and bringing that up with these models into higher layers. And there's also, of course, the ability to take this data and bring it into other systems like an ERP system, like JD Edwards or others. And often that integration with those systems is sometimes through an API, like a REST API or SOAP API or some other mechanism that might be there. Ideally if these tools could also support these tried-and-true open standards like MQTT that we talked about, then they can consume that data naturally with that context right away. That would certainly make this world a lot simpler and I think that's where a lot of movement is going to. It's not just smart devices that are supported. That has a source of the data, but it's also other applications and leveraging an open standard like this allows data to be accessible in many places. But even despite having that, there's a lot of integrations that can happen with the various tried-and-true mechanisms that we can do today.
50:49
DP: Thanks, Travis. So I think I'll throw this question to you, Arlen. "Where is the sensor configured? I'm guessing that you are connecting to a LoRaWAN stack that has been configured some place." That's David's question, Arlen.
51:02
AN: Okay. Well, full disclosure, that device that was running Ignition 8.1.1 Edge was a multi-tech device and that multi-tech device already has a LoRaWAN server in it as well as a joined server. And what we did was we took the definition of those binary things you were talking about, those cryptic LoRaWAN messages, and those are being parsed at the lower level within the stack and being populated into those UDTs. So for all the sensors that we know about, the Radio Bridge sensors, the Sushi sensors, the Laird sensors, those are already done. So as other sensors come out, we'll be doing that integration as well, and hopefully that means that... I know a lot of the LoRaWAN sensors are making people connect over the Internet to a back-end and then pay to get their data back, which in the sector that we're in, that's pretty much untenable, and so now we've got the ability that multi-tech gateway that I just demoed was not connected directly to the internet, it was only connected to our secure MQTT infrastructure.
52:20
DP: Thanks, Arlen, here's another question. A couple of people have this question, one says, "Is there a similar integration capability in Azure?" Another one said "AWS SiteWise which is demonstrated by Azure... Any similar capability with Azure IoT available or being worked on?"
52:34
AN: Yes, the same, Azure has time series insights, which is a very similar service to Amazon's SiteWise service, so we are working on that, hopefully second, third quarter availability this year.
52:53
DP: Cool, and another one, is it possible to integrate with AI decision-making tools?
52:58
AN: One of the cloud formations that Amazon has is that they already understand the models that are in SiteWise. So they have cloud formation models that will pull that into S3 buckets that can go directly to SageMaker. So they are starting to get the tools in place that once you populated your model with the measurements within SiteWise, then you can start leveraging all of the other tools that Amazon analytics has available.
53:32
DP: How do you avoid data security issues related to this data access? Have you heard about Zero Trust?
53:38
TC: Security is of course paramount with all of this, and it's important to at least note the underlying technologies that we're talking about here, say Ignition Edge as an example, that's gonna communicate at the edge of the network to those polling protocols right there, so that's gonna be local, a lot of times, these machines are dual NICs, so there's one NIC that talks to the PLC and then the other one is What reaches out to a higher level system or the cloud. And with that, with MQTT, the connection is gonna be an outbound connection, there's no ports that are open on the firewall inbound, which is really important from a security standpoint, it's over a TLS secure connection, at least that's what you want to use. So it's an encrypted connection, and with MQTT, there are access control lists and guarantees that we can ensure that data is read-only or sent up or only accessible by the right parties with a lot of this stuff. So there's a lot of security around all of this and definitely where a lot of people who are looking at these services like SiteWise and all of that, are really just a matter of pushing data up and leveraging that data further with no way for that control to come back down.
54:51
DP: Thanks, Travis. So I'm gonna ask one more question here and then give you guys sort of a wrap-up question, as we'll come to the end of our time. And I repeat, we won't get to all of these questions, there's a lot coming in the queue still, so this question from Tom says, "This looks good for asset tracking, but how does this work in a discrete manufacturing line with a lot of relational data?" So either Travis or Arlen, take a shot at that one.
55:14
AN: Travis I'll let you take the relational data part of it.
55:19
TC: The data models that we showed here today, some of them were a little more complex than others, but there are definitely bigger, more complex models that are out there. I mean, fundamentally understanding how we're going to design and building these things out properly is, I think is a very important thing to work with, and a lot of the processed data could easily be modeled that way, there's also data you can get from other sources, relational data and what-not too, that you could bring in that you can model, but it's really about standardization and about trying to encapsulate the hierarchy in the objects that are really important for what you wanna do with that data, and providing the right context around it.
56:06
DP: Thanks, Travis. So listen, we're coming to the end of our time, but I just have to have one non-technical question that came up here, maybe I'll throw this one to you, Travis, and then give you a chance to say anything you wanna say as we wrap up, Arlen. This question relates to the... We talked early on when I mentioned this is a... DataOps is a methodology, it's not some exact solution or piece of hardware, so the question is, any suggestions about getting started with the people requirements of DataOps? What if some people in the company are resistant to democratizing data? What types of databases does Ignition work with? That kind of overall getting started question to you, Travis, and then just a final wrap-up from you Arlen.
56:47
TC: Okay, yeah, that's a really good question. I mean, that is one that fundamentally does have to be answered, and to look at within your organization, how everybody sees and wants to approach it. I mean, fundamentally, here's where a lot of the problems happen, is when projects are driven from top-down and kind of like "this is an order, we gotta get this moving" and it's harder to get people to have the buy-in, people to truly have that buy-in. But fundamentally, if you could bring more people together, explain the value that's gonna be brought here, and also do it in a way where they're not compromising anything on the OT side of the fence. I think this is where a lot of the riffs happen, it's like, well, I don't want to jeopardize my OT system, I don't want to make it fragile or I don't wanna break it, these kinds of things. We could demonstrate this kind of functionality with having a better superior system on that side and providing value to everybody. There's value that you get just by being able to democratize the data and provide these models and leverage it. Not only just on the OT systems, but of course at the higher layers as well. But getting them to be a part of the overall solution to see kinda where the company wants to go, see the value that can come out of it, what's the return on investment that's there.
58:08
TC: I think a lot of that is where you can start getting that acceptance and the willingness to go further, plus there is gonna be an investment with this, sometimes adding Edge Computing in place, or there's just implementation costs to build those models, they don't already exist, I mean, there's definitely commitment that has to happen, but there's gotta be a reason to do it. There's a lot of things you could do just on-premise and be able to just get data to infrastructure more accessible, even just within the organization, especially to the business side that's very valuable, but also leveraging the cloud, you gotta have a reason to do it. Some people are, for example, predicting machine failures or tuning processes or those kinds of things, because they have a real need and because they can save money, time, whatever it might be. So having a clear vision of where you wanna go and getting all the players to buy-in, by getting them involved in the process and really being able to demonstrate that you can have a better system, and be able to get the data is really important here.
59:08
DP: Thanks, Travis. So Arlen, one minute wrap up, before we end off, over to you.
59:13
AN: I'll just back up what Travis said, we've said this from the beginning, this is not IT down to OT, this is equipping OT with tools to build a better operational infrastructure that's cloud ready going forward.
59:28
DP: Thanks Arlen. Arlen, Travis, totally appreciate your time today. Very, very insightful presentation and demo. For those of you on here, our next webinar is scheduled for March 25th, so please mark your calendars and stay informed about all our events through social media, our weekly newsfeed and our podcast. Thanks so much for joining us today and have a great day.
Want to stay up-to-date with us?
Sign up for our weekly News Feed.