Changes Towards The Digital Transformation - Turn and Face The Strange
46 min video / 38 minute readSpeakers
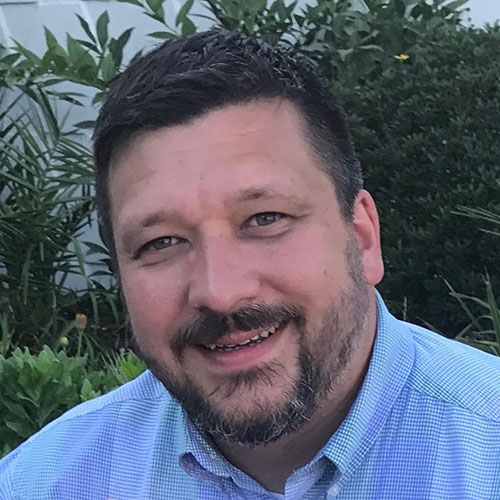
Peter J. Photos
EVP Engineering - Chief Scientist
Streamline Innovations
While data acquisition systems at the process level have become increasingly universal, the true Digital Transformation vision – the integration of all data across an organization to higher levels within a company - still faces a number of hurdles around bandwidth, multiple data-entry points, and conflicting software platforms. Here, we present how SCADA-driven data via Ignition with Starlink Satellite-Based Broadband can be combined with manual-entry mobile Perspective applications to provide a rich data source at the field and operations level. Once in the cloud, that data, in turn, can then be combined through API-based integrations with third-party platforms to provide higher-level insights to Research, Business Development, Engineering, Financial, and Executive divisions. Thus, from Operator to CEO, Ignition provides a true data integration platform up and down an organization.
Transcript:
00:03
Kristin Bainbridge: I'm Kristin Bainbridge. I'm a Sales Representative here at Inductive Automation and so I'll be your moderator today for this session, “Changes Toward the Digital Transformation - Turn and Face the Strange.” So to kick things off, I'm gonna introduce you to our speaker today, which is Dr. Peter J. Photos. So Peter is the Chief Technology Officer at Streamline Innovations and has 20 years of experience in product development in oil and gas engineering. He is an expert in chemical engineering, chemical additives, the OT/IT convergence, and process control. He's also an author of over 20 patents and patents pending. Peter earned his undergraduate degree in chemical engineering from Princeton University and his master's and PhD from the University of Pennsylvania in chemical and biomolecular engineering. So please help me to welcome Peter.
01:25
Peter J. Photos: It's the title. So thank you very much. Welcome everyone. I'm talking a little bit today about where we are and where we're going, and I used that song “Turn and Face the Strange” because things are changing and I want to talk a little bit about what is possible in the next five to 10 years in terms of automation and process control. But I'm gonna start going backwards and start with talking about the industrial revolution.
01:56
Peter J. Photos: So we are on what's been called the Fourth Industrial Revolution. We're entering the age of imagination. We started, 1760 was the First Industrial Revolution, that was the agricultural revolution where we went from physical energy to mechanized energy. And then we went ahead a hundred and change years and electricity came out and we came across the electrical revolution and we entered the third industrialization, the, oops, the Second Industrial Revolution, the electrical age, when we started to use electricity to help us.
02:35
Peter J. Photos: And profit became and technology came out of electricity. Some of you will remember the third digital or the Third Industrial Revolution, which is the digital revolution. And some people still haven't adopted it yet. But frankly, I'm not sure if the Renaissance has been adopted by everybody yet. So the digital age is the Third Industrial Revolution. And I pinned it back a little bit further 1962, that's when we started to use computers to do these calculations. But we're entering the Fourth Industrial Revolution, and that's the imagination age because it's no longer about what you're capable of doing, it's about what you're able to imagine. And I'm gonna give an example: DALL-E.
03:21
Peter J. Photos: Think back a hundred years ago. If you wanted to make a picture, you would have to hire an artist of the highest quality who would have to do something, who would spend half his life studying the mastery of painting, but also how to make paint and how to make brushes and all the associated things that go along with becoming an artist. And then we get to an age where you needed a photographer and in their first photography required understanding to develop your own pictures and understanding the chemistry associated with that as well as lenses and shapes of that. And then it became, well, you just needed to buy a camera and some film and you needed to develop it. And then digital cameras came by and boom, you take a picture and you go. And then, but still, there was still artistry there, there was still Photoshop, there was still construction, there was still things that you needed to do.
04:12
Peter J. Photos: Today we live in an age where I literally can go to a computer program and type in “two chickens riding a motorcycle over a bridge that looked like stuffed animals.” And I kid you not, that picture gets generated. That's not a search engine. Most of you know DALL-E, that's not a search engine, that's not someone searching for that image. That image was created for me by this text in 30 seconds. Pretty close. I was demonstrating this and someone said, “Pick pink elephant sitting on a refrigerator in the style of a New Yorker cartoon.”
04:49
Peter J. Photos: Boom. There's actually three or four DALL-E images in this presentation and I challenge you all to find which ones are and which ones are not, because it's not easy. These are not search engines, these are generated images based off of those words. If we can create a software that can do that, what can we do from a process control and automation standpoint? We could easily go where no one has gone before or go gentle into that good night. Where is automation going? And that's what I'm gonna ask today.
05:24
Peter J. Photos: I just need to understand up and down though. So if we go from this example, two chickens riding a motorcycle over a bridge in the style of stuffed animals, what would be the equivalence in process control or automation? “System controlled by maintaining liquid in this vessel at all times without spilling.” That's kind of scary to say that, but again, “reaction rate kept at equilibrium.” I really don't know what that means. “Run unit under optimal conditions.” Can we say that? Can we get to the point in life where we can just write that to the computer and the unit runs?
06:07
Peter J. Photos: And I'm gonna tell you that's just at the process level. We're gonna ask that question in deeper in a minute. And the reason and the way forward to that is really a very logical progression. It's going from programming problems, to configuration problems, to autoconfiguration. And I want to use an example of home automation. Okay? So some of us geeks, remember in the late '80s, RadioShack selling the X10 platform. This was really cool. It was radio controlled switches. So you have a box that actually looked like that right there. And it would send a radio signal to boxes that sat in your outlet or in your light switch, and you would program the channel in which it would communicate. And you could hit the button and you could turn the lights off and on in your house, and then you could hook it up to a rather small computer with a rather small screen.
07:02
Peter J. Photos: And program it. You can make all these programs in it, but it's all programming, right? You had to understand RS-232 and you had to understand RF and you had to understand channels. And you had to understand a lot of components in that to make that work. Well, okay, fast-forward 20 years and we now have Alexa where we plug things in and go. Now it's configuration issue. Now you gotta name it, “bedroom lamp” or you gotta name it, “living room television.” Okay, we gotta set some configurations and we can make it go. So we've gone from a programming problem where you had to be expert at it to a configuration problem. You see the parallel with artistry. We've gone from having to know how to make paint and brushes, to picking up your, dropping off your film at Fotomat.
07:56
Peter J. Photos: Last step is autoconfiguration. So now the next step is, I bring a light, put it in my house and I don't need to name it. I don't need to. I just walk into a room and say, "Turn on the light. Turn off the light." We're not there yet. But suddenly that leap of faith, that jump, doesn't seem unreasonable to anybody in this room. You are all thinking about how that can be programmed and automated. So going from here to here seems like a huge jump, but going from here to here didn't seem bad. And going from here to here doesn't seem bad. So it's the same story about DALL-E. It's the same story about process control. It's the same story about home automation. It's not these big, these jumps are manageable, but if you think about the overarching story, it's huge. But why is process control and why are automation still stuck in the dark age? Well, process designs are wholly driven by mechanical engineers, not programmers.
08:54
Peter J. Photos: So the mechanical engineers drop the P&IDs, they hand it to the process control engineer and they say, "Go, have fun. Call me, when it's designed." And so process control is still the tail being wagged by the dog. And so few industries have decided to go ahead with cross, with advanced model predictive control or AI. A mechanical engineer would never let you say, "Well, we're just gonna run the unit at equilibrium." That doesn't make sense. So, because that's too scary because it's not the... The mechanical engineers are running the show, not the process engineers. And automation is still basically sequencer steps. We're still in the programming phase. We are still a programming problem. And that's the challenge is we can't get to autoconfiguration 'cause we're still in the first stage. We still have to design our own brushes and our own paints. But the technology's already there. If I can get two chickens riding a motorcycle on a bridge as an image, why can't I get something decent in SCADA, right?
09:57
Peter J. Photos: And this is the thing, a lot of these tools are here, right? We all done most of these things, right? Safety/interlocks, the idea loops, manual inputs, alarming, real-time KPIs. As we get to the bottom, they get a little more complex. But a lot of these things we've done, soft sensors, most of you have that. Python scripting, okay? Most, some people. Configuration problems, alright. Well, OT standardization, HMI templatization, containerization, we just heard that talk a few hours ago about containerization and where we're going with that. And virtual imaging of systems.
10:32
Peter J. Photos: And finally these are not unreasonable steps. And then as we get to the fancy cool stuff, fallback algorithm, fallback algorithms, predictive maintenance. Okay? A few of us are doing this. Self-tuning PID loops, I'll keep talking about that in a few minutes. Machine learning. Okay, now we're, this is where we gotta get to. So we have to get from the top right to the bottom left. It's a long journey, but someday I'll figure out the remote control. So I'm gonna talk a little bit since I was paid for by Streamline to come here, I need to give them their five minutes of ad space.
11:10
Peter J. Photos: I am CTO of Streamline Innovations. We are a company, we're about six years old. We started in 2015, 2016, and I'm gonna talk a little bit about what we do. And what we've done is we are kind of an odd duck for this conference 'cause we're not integrators and we're not end users. We are both. We actually integrate it, we design, build, integrate, operate, service, and maintain gas processing plants. And these plants are roughly the size of this room. They treat what's called hydrogen sulfide. And the story is an interesting one because the technology we're doing is actually developed in the 1930s and it's been commercialized in the '80s and '90s and some to varying success.
12:00
Peter J. Photos: And we started five years ago. So we're late in line. And the challenge is this, and this is why this is important. Liquid redox is a chemical reaction, two chemical reactions, that remove H2S from natural gas. Don't worry about that. But they need to be maintained in a very exquisitely narrow band. So that means we have to do two chemical reactions, maintained in real time. And maintain that in a small band, 24/7. Now, let me explain a little bit more about this. First of all, hydrogen sulfide is an extraordinarily toxic gas. Right? One part per thousand, one breath, will kill you. Okay? Super toxic, super flammable, super dangerous. It's also found in every gas well in Texas. It's found in about 40% of all gas wells in the world. So obviously, you can turn your stove on if you have a gas stove. You don't want H2S coming out. Yes, you can burn it, but it turns into sulfur dioxide, which makes acid rain. So it needs to be removed. Okay? And to make it even worse, it's not just found in oil and gas. It's also found in part of hydrogen generation. It's found as part of farms. I'll talk about that in a minute. Your homes produce H2S.
13:33
Peter J. Photos: Everywhere where there's biological waste, there's H2S. As part of power generation, it's H2S. H2S is everywhere. In every wastewater facility, in every toilet in America. So it's everywhere, and it needs to be removed, and this is a big challenge. And other people are burning it, which will create SOx or NOx, which pollutes the environment. And our way, we actually convert it using this process, to elemental sulfur.
14:01
Peter J. Photos: So again, not to give the chemistry lesson, but elemental sulfur is also known as fertilizer. So we actually take this terrible gas and convert it to fertilizer. And we have to do it by maintaining a chemical reaction in a very narrow band, in a very remote area, with 24/7 operations. And by the way, the gas flow rate does this over time, and the H2S level does this over time. So you've got two variable inputs, and you're maintaining a chemical reaction with a very narrow band. And you need to keep constantly adjusting this. And you're in the middle of nowhere, with no bandwidth, and you have to keep your costs down because this is all based off of operational efficiency, and you only have about $50,000 in your entire OT budget.
14:46
Peter J. Photos: Bit of a challenge. But that's how we did it. And ultimately this is the process flow. I don't need to go through, give you a chemistry lesson, but it works. But the challenge is, it needs to be done as efficiently as possible. Because in the old days, they would build something huge the size of this building, they would hire three people to sit there and watch it, and they would maintain and operate the unit. Works great. They did it all the time. And our ancestor company that came before us, that's what they did. But we wanna go smaller. We want to automate this. We want to make this thing work. 'Cause we wanna go smaller and smaller, and I'm gonna get to the smallness in a second.
15:26
Peter J. Photos: And we automated it. We went through all of this. We did all the process control, all of the automation we could do. Anything we go back, we could try again and again and again. And I talked here three years ago at ICC, and this is the slide that I had where we had three unmanned plants in south Texas, seven under construction, which was amazing, one manned plant in west Texas, a couple of wastewater treatment facilities, a couple of things in New Mexico, and one million pounds of sulfur treated and 99% uptime.
15:58
Peter J. Photos: That was three years ago. Okay? Today, we have 27 units across four states operating. We're averaging one new unit a month. We have installations across Texas, New Mexico, Illinois. We actually already installed the one in California, and they're just waiting to start up. We have treated over a billion gallons of wastewater and over 20 million pounds of sulfur dioxide removed from the atmosphere. We started landfill operations in 2021, and here's the zinger. When I had this slide, I didn't put the number of operators Streamline employed, but it was eight. Three years later, 27 units later, we have eight. That's scale. How did we do that? Because we automated the crap out of those units, and we still have the 99% uptime. So, if anyone ever tells you automation budgets are not worth it, show them this slide.
17:05
Peter J. Photos: Just to hammer it home, because I'm going to use these slides as a reference later, some of the clients that we have you've heard of, some of the places where we treat water you've heard of, some of them you haven't. We've got dots all over the map, and we have a variety of different products. What makes us different? Yeah, our chemistry is different. Yeah, our business model is different, but that's why we did it. I'm gonna show you just a quick project that we did to get down to the meat and potatoes. This is a facility we built in Pecos, Texas. What's really cool is when we built it, they were communicating across a Tropos Network, on DBD Tropos Network. They have since moved to a private LTE network, which is super cool, by the way. But they're using a private LTE, and we operate admission off of that private LTE network. This is two different clients, but they're integrated into one. Typically, there's three sites that look like this, so you would need roughly six full-time operators to maintain that after you operate this, and we wanted to do this with three. Since I just like to get to results, we automated it. We took the same principles we took to that small unit, and we built this plant. And we have artificial intelligence and model predictive control running in the background. We have daytime operations.
18:31
Peter J. Photos: We have one guy that operates all three plants, two guys that operate all three plants, and then nighttime, it's ad hoc. So, we did it with two FTEs. No call-outs. This is a 24/7 monitored remotely, operated remotely, predictive maintenance, and a 99.5% uptime. So these are two of our FTEs. But let's go even smaller, but that's the advantage, 'cause we automated this. Now we're CapEx driven, not labor driven. So we can go even smaller. So let's talk about poop to power, #PooptoPower. Biogas. Biogas is taking animal waste, manure, food waste, anything, cheese processing plants, biomass, you put it into a digester, you put it into a swimming pool, you cover a balloon over it, and you let it rot. And as that rots, that bacteria gives off methane. Methane is also called natural gas. But it also gives off CO2 and it gives off H2S.
19:35
Peter J. Photos: More importantly to me, it gives off H2S, every living thing contains about 2,000 ppm sulfur, even you guys. So if I took you and digested you, you would give off 2,000 ppm H2S. That means every farm in the world can be a power generator. It can generate natural gas. We're in California, you'll appreciate that, but let me go into geopolitical behavior, comment for a second. Europe. Russian gas from Europe being cut off. What's the next source? Libya. What's the next source? Cow. So H2S, methane, biomethane, which is a purified version of biogas, is a huge opportunity in this world. Plus, it's as green as you can get. We're literally taking poop and turning it into power. So keep in mind that 2,000 cows are equivalent to one small gas well. To give you an idea of the metric, so there's a lot of power in this world that can be generated from cows. But we need to get through all of this and we need to treat H2S most importantly.
20:45
Peter J. Photos: And now it has to be really, really small. So what did we do? We went smaller. So this is actually our Valkyrie Eco. That's actually what it looks like. That's the first one we built. That fits on a 15-foot trailer. That is a big pile of pig poop that's being digested. The gas comes out of that, goes through a compressor, goes through our unit, and then it goes on to become the burner. It goes to a cogeneration plant. We built a shipping container-sized unit, fully automated, remotely operated and monitored, low-cost, and it's refinery-level control. Now think about how challenging this is. We're getting down to something the size of a shipping container that's a half a million dollars, and we're operating our unit on that. It must be smart. So let me ask, how do you make things smart? I'm going to go back to humanity, because the best thing we could do is be anthropomorphic. Our own brain. There's four major sections of the human brain. Even though it's called the triune brain, there's four sections. Don't ask me why. I guess doctors didn't know Latin. I don't know. But the triune brain model is there's four sections of the brain. The first and oldest is our fish brain, your amygdala.
22:19
Peter J. Photos: It's the evolutionarily oldest part of your brain. It's the part that survives. It only does four things. It makes sure you food, flight, fight, and orders. Alarm, it makes sure you're alive. It makes your heart beat. It makes sure that your heart is beating. It makes sure that you're alive. It makes sure that you get out of trouble. This is your fight or flight routine. The next one is your reptilian brain. It's the lowest level of your brain back here. Your hindbrain. This is your instincts. This is what controls your breathing rate, your heart rate. This is what makes sure that you don't instinctively control your breathing rate at any given moment. You don't control your heart rate at any given moment. This is making sure that everything is operating, your metabolism. Your food is being digested. You are eating. You're sleeping. Everything is operating as normal. Think about this from a process control standpoint in a second. The next one is your mammalian brain. This is what makes you a mammal. You have feelings, emotions. You can have control over different parts of your body. Limbic system. Memories, interactions with others.
23:34
Peter J. Photos: This is your mammalian part. And then finally, your human brain. Your neocortex. This is the most advanced. This is the most recent development. This is what makes you human. Rational thinking. Higher level of thinking. Language. Imagination. Now we're going to take this model and lay it over a process control system. And we talked about the amygdala. And, you know, fight or flight. This is an ESD button. The reptilian brain. Making sure that everything happens the way it's supposed to every second. Your heart keeps beating. Your breath keeps going. What does that in process control? The PLC. Good, reliable, and dumb as a brick. Next slider is your limbic system which is communication. Making sure everything reaches out. I actually have a computer there, but I meant to put Ignition there.
24:31
Peter J. Photos: This is your SCADA system in your body. It's making sure every part of your body is connected and communicating. And you know where your fingers and toes are. And then finally, your neocortex is kind of this highest level computer. This is your AI Watson-level computing. That is at the highest level. And when you think about it like that. And now you think about how your brain works. Well, now you just got to assign the right task to the right job. Because up here you've got brains and down here you've got brawn, so to speak. So it's brains versus brawn. You've got more processing speed up here. You can take longer time to acquire data. And down here you have faster bandwidth. It needs to be faster processing time. And faster time to respond. And so when you think about it that way, now we can start thinking about the right tool for the right job.
25:20
Peter J. Photos: And we're going to brain, broad brain, which is Linux with best computer programming, handling data, large amounts of data but doing it efficiently, brawn, it's just about turning through the huge amounts of data and ensure that everything works all the time. So if we take out all the jobs that we'd like to do and put this on this kind of matrix of data acquisition, how might we... How much data you need to acquire and how much computations you need to do, we can start talking about all of the things that we'd like to do in process control. An ESD for example, it's a button, you hit it, turn it off. It should be super fast. So there's no knowing acquisition time and no computational requirements. Interlocks require a little bit more. As we get up here, we start seeing things like fuzzy logic and data inputs, and then we get up here, we see model predictive control, and we get all the way up to the top and we have machine learning. But over here we have things that don't require a lot of computation, but huge amounts of data, like real-time P&L. And so we can lay out all of these things and compare back to what I just talked about, using the right part of the brain at the right time, and we can start laying over different pieces of where they can operate.
26:33
Peter J. Photos: So down here obviously is where your PLC exists, where it's short amount of computational and short amount of data requirements. Out here your edge computer needs more power but it's not gonna hold onto data for very long. As you get up here, you get AI servers over here is your enterprise management system. So you can start laying out the data and start thinking about how are these things going to work together? So once you got everything laid out, now how do they talk?
27:02
Peter J. Photos: Well, they can just start laying in different families. OPC UA is how a PLC talks to an edge computer, Ignition gateway APIs, all of these things start coming into place and you start laying this out. Okay? From here I'm just going to do a topological shift. So keep everything in all the arrows and all the pieces of equipment here, but let's just rearrange the furniture so it looks a little clearer. And so our edge systems in the middle, you've got the edge computers, you've got all of these other things sticking out and out of the center and into the center is your edge system. So choosing your edge architecture, choosing your edge device is extraordinarily important. #Ignition, they can pay for my... They're paying for my dinner, so I give them a shoutout. Now, but honestly we use Ignition as our centerpiece and I'm gonna go a little bit into our architecture. Our hardware we use is Stratus ztC Edge, which we use with redundant or single node depending on the size of the unit.
28:04
Peter J. Photos: We put virtual machines inside, I'll talk about that. We then go to Starlink, which goes to the cloud and then out up to Azure. And honestly, we're actually thinking about changing that. And then finally ends up in Dynamics. So our Stratus machine is in the center. This is our edge computer, obviously the PLC to the unit, Ignition, we run full-blown Ignition, by the way. We have four virtual machines running on Stratus. One is Ignition, one is PostgreSQL, one is a Python Scratch script server, scripting server, and one is Studio, Windows, which is running Studio 5,000. So this has given us an incredible flexibility because we have a local HMI, we have local historians and all that happening, good stuff. We have Appgate running, which gives us cybersecurity connection back to our system. And then our guys in the field can actually just connect to the system, to the Windows box, running Studio 5,000, check out our license, and it's like they're have a computer sitting on the unit. So we could troubleshoot and operate the unit at all times just through this machine. This is the cool, sexy thing we've done, which is Starlink. Starlink has been a winner for us lately. We have... I'll get to that in a second, and then in the cloud we can use some higher level analytic tools to get up there and do those calculations.
29:28
Peter J. Photos: But what else can we do with Ignition? What are some of the cool things we do with Ignition? So we have not just data coming in, but we are data hungry hungry hippos. We then bring in, Stratus actually has an API that connects to Ignition. So we can pull data about the computer we're running on into Ignition, and we break that data into the system. We can track CPU and memory. The modem has data coming in. Starlink has an API that lets us connect to it, that connects to Ignition. That also gives us the ability to restart these pieces of equipment through APIs. But then we're doing things like a script is running, pinging the National Weather Service, pulling in the weather forecast. Another script is pinging Google and checking the ping time. And we monitor and historicize the ping time. So we have full visualization into our unit at any given time, at any moment. And we have 27 of these. So this is a tremendous amount of data being acquired locally and then that gets transmitted up into the field. So Starlink has been a godsend for us. We actually have four units operating right now. And that's just in the past three months, we got Starlink out there two weeks after they came out. And we are actually seeing remarkably better improvements.
30:48
Peter J. Photos: And honestly, it is in that panel somewhere, yeah it's just a little white box sitting in there, and then that's the antenna, and that's a really super cool antenna that's got a gimbal in it that actually finds the satellite. And we are going to have Starlink on all our units by the end of next year. As I mentioned, we do weather forecasting, we shut down for tornado warnings. These are trivial things from a process control, from a programming standpoint, these are trivial things, right? I mean, how hard is it to know if the local area you're in has a tornado warning, if you have an Internet connection? I mean, my phone goes off all the time, if we had an Amber Alert in the middle of this meeting, you would all see it. So why is it that difficult to understand that a unit can shut down in a tornado warning? It's not. But from process control standpoint, it's mind-blowing. Non-linear calculations, we do fast Fourier transforms on our vibrations to monitor our pump behavior. Again, simple stuff, but we do that at the edge. As we get fancier, we started to do some non-linear regression analysis on some of our predicted values, and we're trying to find how we can land, how we can predict without using certain instruments, and then we can tell if that instrument is working or not. So we're doing a...
32:07
Peter J. Photos: The word escaped my mind. An algorithm with two computers where we're doing a competitive algorithm to make sure that these instruments are operating the way they're supposed to or whether or not they need to be calibrated. So, really cool stuff and happy to answer questions about those. But I want to ask the question, how do we go even further? I started this talk by talking about how far can we go?
32:34
Peter J. Photos: So we have the tools, we saw some things we can do, we know some things we can do. How far can we go? These are the oldest footprints of humans in North America found, and that's a slightly more famous footprint. How do we go from there to there? Well, we do baby steps, baby steps. But beyond SCADA, how do we move an entire corporation beyond that? And that's where I'm gonna, this is where I'm gonna kind of talk a little bit more about some other things that you could do.
33:03
Peter J. Photos: So I'm gonna do data flow for dummies or executives, which are the same thing. You know, data starts at the instrument level. There's data here, tons of good stuff, that's a screen capture from the instrument to, by the way, this is also what our HMI looks like. So you also see a sample of that. But to the field operator who sees an HMI to the lead operator who sees a simplified HMI so he can monitor multiple lines.
33:34
Peter J. Photos: To the control room, by the way, that's our control room. Twenty-seven Ignition screens all running simultaneously. That also uses a hypervisor screen where the data gets acquired. Okay, so we started with the instrument, moved up to the control room, to the chief engineer level, who, now this becomes a chart where we can do a health check on every instrument, every unit. These are now units and there's 27 of them.
34:04
Peter J. Photos: And these are key KPIs for each unit that we can monitor in real time and see the health and the color check. And I know this unit's running well, this unit's struggling a little bit. See these units and we can review that once a week. I, and my team, go over this with the operations team and say, you know what, we need to change this a little bit. And this is a little concerning. That's okay because that's alright. These units are running great, this one’s not too well, do something about it. It is moving up.
34:34
Peter J. Photos: Now that data can get consolidated and we bring in our ERP and we bring in our labor, our work data, and we actually generate data that I've been asked to blur this for security reasons. A real-time PNL of the company, which actually makes it all the way up to the CEO.
34:52
Peter J. Photos: So literally this data switch starts off at an instrument that we had to make sure we had the instrument working and making sure that's thing makes its way all the way from the bottom, all the way to the top of the company. And that's super important because that means changes could be made and we can get from one, we can pivot as we need to based off of this data, which we know is live and fresh and ready and all because it came all the way from there.
35:24
Peter J. Photos: And every person along the way is checking what they need to check to make sure this data, as it makes it up way the value chain, gets to where it needs to. And that's where it gets fun because we're taking granular data all the way to strategic. So I come back to the first question, where are we going with automation? Because I said, you know, showed you DALL-E.
35:49
Peter J. Photos: I showed you some things we could potentially say to a process engineer on where automation's going. And I'm not saying that we went from all of these wonderful things about Streamline because only because we did this. I mean, fortune and chance happened to them all, but this certainly helped, right? But that's not the point. The point is, is everything I showed you here, all the way up to here is still just data, is still just subject to the same rules of automation and imagination as this.
36:33
Peter J. Photos: And so at the instrument level, we think, how do we make that smart? What's our imagination think? Make this valve open, simple. We can do that. At the process level, make this unit run optimally. Which you guys rolled your eyes a bit at it, but not totally off the wall. The control room, automated control room. An iEngineer, at the executive level, an iExecutive, an iCEO, how far can we really get to the top here?
37:06
Peter J. Photos: What's holding us back besides our imagination? And a good DALL-E image of a boardroom of robots. So that's where I want you to imagine the world that goes from where we just kind of do what the best we can to where it is just limited by your imagination. And if you want to think that I'm totally off my rocker and that's totally impossible, I want to remind you one thing that Starlink was almost called Skynet.
37:37
Peter J. Photos: And I don't need to let anybody in this room know what Skynet is, but you all know, so it's not that far off. So with that, I'll leave that open to questions and perfect timing.
38:00
Kristin Bainbridge: So if you have any questions, I'd love it if you could use the microphone. Otherwise I will have to repeat it. Any questions out here?
38:12
Peter J. Photos: So, last of the...
38:15
Kristin Bainbridge: Yes.
38:15
Peter J. Photos: Conference. So everyone's got fatigue.
38:16
Audience Member 1: Thank you for sharing the architecture that you showed and how you capture the data. You had like another diagram with like, some of your like, components. I'm just wondering how the components are actually sensorized and connected and are you using wireless for example? I dunno how, if you can just, give us a little bit...
38:35
Peter J. Photos: You're talking about that one?
38:36
Audience Member 1: Yeah. So this is kind of like a much... At a much higher level, right?
38:39
Peter J. Photos: Yes.
38:40
Audience Member 1: That diagram on the left represents all of your accomplishments.
38:43
Peter J. Photos: Correct.
38:44
Audience Member 1: Can you get into a little bit more detail how you get the data from your little bit of components sensorized again, in a PLC for example?
38:51
Peter J. Photos: Okay. The question is how do we get the data from the field to the PLC? And what are some of the ways we've done that? There's a couple of different things we've done. The most traditional way is this classic 4-20 milliamp sensors that most of the nice manufacturers will make you that just go from pressure transducers to movement. And we have our fair share of those, probably several hundred. However, there's more data than that that comes along with that. And so there's a, if you're familiar with HART, but HART, which is that architecture communication protocol underneath that. So not only does our data go traditional PLC now, which is 4-20 wired into an I/O card on the PLC, that's the bread and butter of the core of a lot of that.
39:38
Peter J. Photos: But then there is another layer of data. The HART data comes in, and that HART-enabled card on the PLC is then also connected to Ignition, which will allow us to pull in HART data straight to Ignition, which then is historicized and stored. To wit, I got more to add to that. When we do our FAT tests, we do it with a special screen that has the HART screen. Rather than doing the trucks, we could do our entire FAT check through Ignition. But then there's other data sources. There's fog instruments that are Modbus TCP, for example, that also come into the PLC and then get historicized. But some of that data isn't critical to the path. We have Modbus TCP reader in Ignition, that pulls in the rest of that data and gets historicized. Then we got some that have their own web pages. We scrape those web pages. We are totally agnostic to where we pull in data. And some of it's classic stuff, some of it's a little off form. Yes.
40:41
Audience Member 2: I don't see any MQTT being used in your architecture. Are you using MQTT architecture?
40:47
Peter J. Photos: Question is, is there MQTT in our architecture? This is an excellent question. We have gone back and forth on MQTT. We originally started with a classic architecture of Ignition Edge with MQTT going to a cloud Ignition gateway. It worked extremely well. The problem we faced was bidirectional control using MQTT. We switched to an OPC UA connection. So our edge computer has, our edge computer is running full-blown Ignition with a tag splitter that then OPC UAs to a cloud gateway Ignition, which I know is suboptimal, but it does allow full bidirectional control from the cloud, which to us is more important than making it optimally data optimization. To us, our primary focus is making sure that when I hit the EFD button in the cloud, it turns off. And to us, OPC UA as our cloud-to-edge connector is the choice for us. Because of that, solely because of that reason. It were data, we would drop it and go back to MQTT.
41:57
Audience Member 2: One more question. You mentioned that in one of your slides you have a project coming up in Oman. Are you gonna be using Starlink on that as well? Is that something you want?
42:07
Peter J. Photos: That's a fantastic… am I gonna use Starlink in Oman? I don't know.
42:13
Peter J. Photos: It's very funny that it's, these units that we build, the sales team goes and makes the deal and shakes hands and we'll figure out the comms at… the comms is literally the last thing that gets discussed two weeks before we start up. Oh, by the way, you have Internet?
42:33
Peter J. Photos: Literally it is the last thing anyone thinks. And I don't even think they thought about the fact that there might be data firewalls around, certainly around Saudi Arabia for example, there's data firewalls. I don't think they even considered any of that yet. My, they dump it on me to worry about over the next six months.
42:53
Audience Member 2: Sorry, one more follow-up question.
42:55
Peter J. Photos: Sure.
42:56
Audience Member 2: From a networking perspective, right? Considering best practices, I know that it depends on the region of the world.
43:02
Peter J. Photos: Yes.
43:02
Audience Member 2: Is it all wired or is it like there some wireless involved? You get the data from the sensors, do you have PLCs?
43:08
Peter J. Photos: We do have some wireless data. I am actually, so our Director of Automation is a PhD, actually he's all but dissertation PhD in automation. And his PhD was in wireless instruments. He is a huge proponent of that and using it correctly. And the challenge with wireless instruments is making sure that you use, we actually powered wireless. Certain things are wireless just because it's easier for us to set, but it's all powered. We run 24 volts to it. We never have to change the battery, but we don't have to run the wire back. And that also allows us to autoconfigure it. If you change the device, it already automatically reconfigures that device using wireless HART. And that works beautifully, but not everything because if you get too many of 'em, they can start competing with each other. And critical instruments are still wired and so those are wireless.
44:10
Kristin Bainbridge: Alright.
44:10
Peter J. Photos: That was just very high-end.
44:13
Kristin Bainbridge: Do we have time?
44:14
Peter J. Photos: We have one in the back.
44:15
Kristin Bainbridge: One more question.
44:15
Audience Member 3: I just, is it a problem or maybe a problem with the people consuming the data that the renderings get progressively looking more like Excel the further you get to the enterprise level?
44:28
Peter J. Photos: Oh, it's exactly. No, you're exactly right. They do look more like Excel because that's where they end up. They do look like Excel because that's exactly where the data sometimes ends up is Excel ends up being the dumping ground, even though it's pulling from, and it comes down to traditional visualization tools. When you go to a CEO and you open up a custom app, who looks at this like, what is this? When you show him an Excel chart, he just feels more comfortable. Even though this is an Excel chart, these are all database links back to an Ignition database. It just happens to be the visualization tool for the future.
45:08
Audience Member 3: Then if this is probably for the future, the it's this is again where culture fuels technology, right?
45:14
Peter J. Photos: Absolutely. You're absolutely right. Comfort, people will prefer something that's more comfortable to look at even though this has a data pipeline jump that's completely unnecessary because I could have built this in Ignition. I have this fight with my operations side all the time. I could build this in Ignition but we like Excel. C’mon, nobody likes Excel.
45:43
Kristin Bainbridge: Join me in thanking Dr. Peter Photos.
Want to stay up-to-date with us?
Sign up for our weekly News Feed.