Speakers
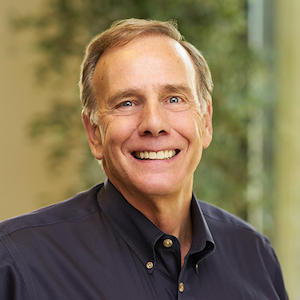
Don Pearson
Chief Strategy Officer
Inductive Automation
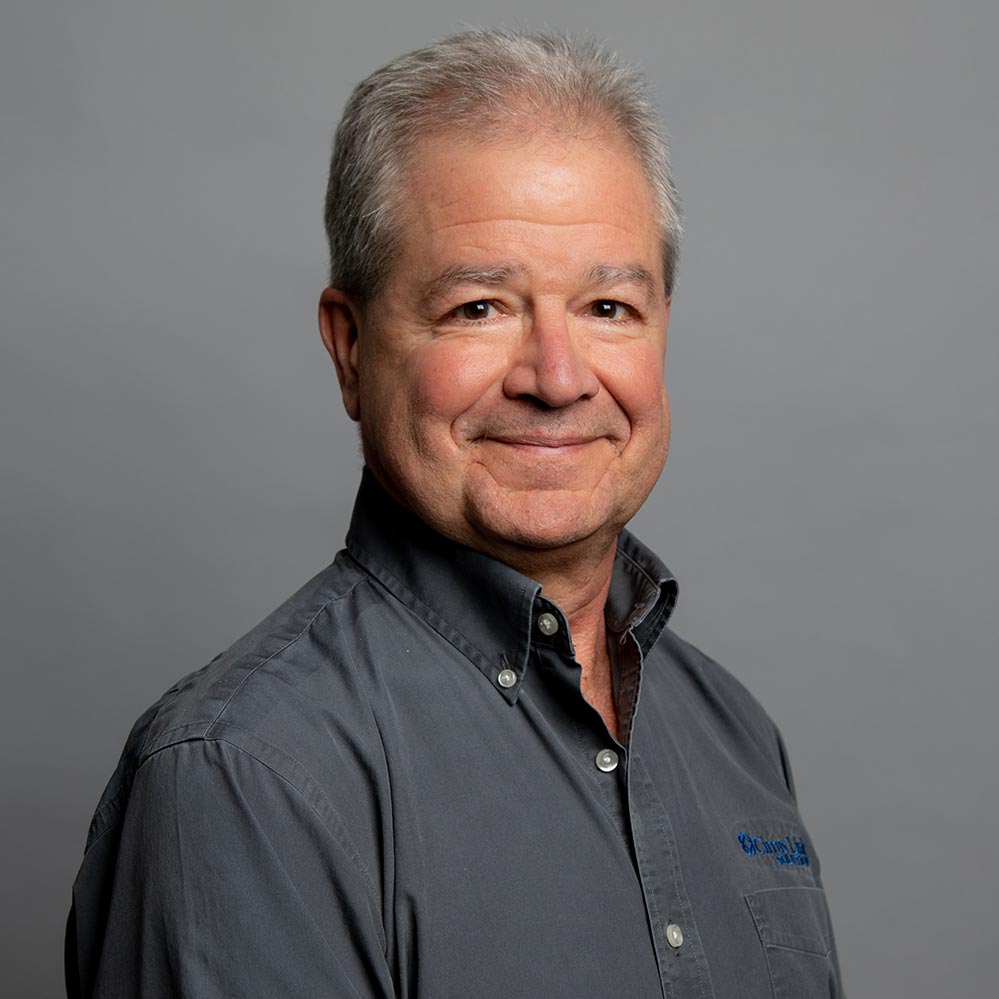
Arlen Nipper
President & CTO
Cirrus Link Solutions
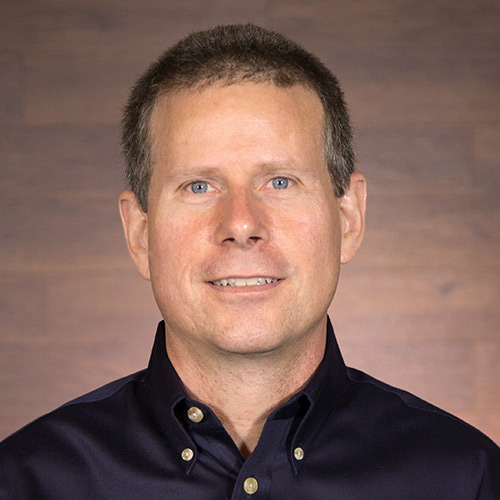
Tom Hechtman
Founder & President
Sepasoft
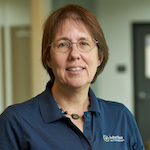
Kathy Applebaum
Software Engineering Department Manager
Inductive Automation
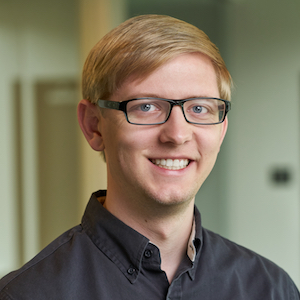
Kent Melville
Director of Sales Engineering
Inductive Automation
About this Webinar
Whether they refer to it as IIoT, Digital Transformation, or Industry 4.0, companies in every industry are looking for a way to get more from their data. However, most are trying to achieve this with conventional architectures and IT-centric methods. The truth is that without reliable connectivity to their operational data, their plans for building a digital enterprise are bound to fail.
In this insightful webinar, experts from Inductive Automation, Cirrus Link Solutions, and Sepasoft explain an overview of the platform and tools you need to gather your OT data and facilitate Digital Transformation.
Learn how you can:
- Publish plant-floor data to a central hub
- Connect ERP and SCADA systems
- Inject tag data into cloud infrastructures
- Improve your process with machine learning
- And more!
Co-Presenting Companies
Webinar Transcript
(The presenter, Don Pearson, briefly introduces Inductive Automation, Sepasoft, and Cirrus Link Solutions, and the panelists, Tom Hechtman, Arlen Nipper, Kathy Applebaum, and Kent Melville.)

The Promise and the Reality of Digital Transformation
6:45
Don: I think we have a pretty important topic, digital transformation. It just happens to be one of the biggest industrial buzzwords at the moment. You hear it a lot, but I think it's important to take a step back and say, 'What does it actually mean?'
6:59
Don: I just want to take a few minutes to try to define what digital transformation really means, and why there's so much interest in it right now.
7:09
Don: The ARC Advisory Group, which is certainly one of the leading industrial technology research and advisory firms, defines digital transformation as you see on the screen. It's the transformation of industrial products, operations, value chains, and aftermarket services, that are enabled through the augmentation of people and knowledge through the expanded use of sensors, data, and analytics.
7:34
Don: That's kind of a mouthful. Tom and Arlen, you both live in this world, I want to ask you what does digital transformation mean to you? Why do you think it's an appropriate term for what companies are trying to achieve now in their organizations? Tom, let's start with you.
7:49
Tom: Yeah, I think organizations as a whole, manufacturing organizations, are challenged with being efficient, really making quality product, and they don't have much insight into their organizations though, in the manufacturing process. It's kind of like trying to monitor 401K with no way to go in and see how it's doing. It gives insight into what's happening in your operation. You can do that because you've got a whole bunch of data down in the field, and you also have a whole bunch of data in other sources, and bringing that together and start making the connections, just to be competitive in this marketplace in this day and age.
8:38
Don: Thanks, Tom. Arlen, your thoughts?
8:41
Arlen: Well, Don, from my experience both, we're seeing a lot of activity in manufacturing. Looking back at my career, if you look at in the 1980s, and then the 1990s and the 2000s, we've got almost 40 years of an entrenched mindset of how we put operational systems together. I would argue that we're leaving probably 90-95% of what could be valuable data, we're leaving it stranded in the field, because today our systems are focused on just getting that operational data. That data that I need to run my pipeline or my factory day to day. But, we're leaving all that other information stranded. I think the challenge here is coming up with the tools and the infrastructure and the technology to get operational infrastructure to the point where now, all of these data analysts, and the experts that I believe will be able to leverage that information, just can't get their hands on it today. And when they do, trying to make heads or tails, get context of that information is a huge challenge.
9:51
Arlen: I think digital transformation for me means not IT down to OT, but enabling OT to provide that information without disrupting day to day operations.
10:03
Don: Sure. Actually, I think you set the table extremely well for this discussion because it really does deal with the kind of dilemma of ‘How does one get started, and how does one really approach it?’ Because, when you think of digital transformation having profound impact on manufacturers, as you said. But, many of them are really still struggling to realize its full potential. Whether you call it digital transformation or IIoT or big data or industry 4.0, industry x.0, I think the point is that every industrial company is trying to come to grips with what this revolution means to them, how to get started and should we start from the IT side, or from the OT side to your point Arlen.
10:48
Don: I think this illustration shows the promise of digital transformation that companies are after, is the ability to get their operational data into all these cloud IT apps and do all kinds of amazing analysis, business intelligence, machine learning etc. Most vendors infrastructure diagrams for their IT cloud, or Edge, or FOB solutions, look a little bit like this. They showcase all of their services, APIs, and they have this little small arrow coming up from the entire OT SCADA, DCS, ICS infrastructure, with a small label that just says operational data comes in here.
11:25
Don: Now, that's nice in theory, but I believe we have an elephant in the room here. Most organizations are trying to drive digital transformation from the top down and their projects don't work the way they're supposed to. Users become disillusioned with the sort of idea of digital transformation. They end up in that Gartner life cycle trough of disillusionment if you will.
11:51
Don: I think it's important to look at why is this happening. Here's what most companies don't really recognize as they develop out their solutions in this arena. The reality is that if you want big data that's going to be required for digital transformation, you have to have big access to that data. You can only do amazing things with your operational data if you have fast, reliable, comprehensive way to consistently capture all of that data and transport it into the cloud.
12:23
Don: Big data analytics with 95% of that data stranded in the field, as you were saying Arlen, is hardly going to accomplish the goal these companies are looking for.
12:35
Don: I guess the question is, why doesn't IT-led digital transformation work? Here's a few of what I think are big reasons. It really doesn't account for the knowledge, skill, time, and effort required to understand to get access to each customers unique OT system. For example, if you spent months developing a machine learning application, but the end of deliverable there's a bunch of Python code with hard coded process variable tags. That means every time an automation engineer needs to add or change a SCADA tag, they also need to modify some Python code. Try to scale that out. It just doesn't work.

13:15
Don: Also, many of cloud and FOG architectures are IT centric. They all require writing applications in a programming language. Basically, they're trying to put coding on top of operating systems and they lack native tools that can be effectively deployed in a digital transformation project targeting OT infrastructure.
13:35
Don: Also, none of these IT Edge products are promoting a better OT infrastructure. You can talk about analytics, you can talk about machine learning, AI, all you want. But, if this solution doesn't work better for that operator in the field, the one who has to run 24 by 7, 365. I'm sorry, it's going to be a nonstarter. What happens is a lot of companies are trying to enact digital transformation by starting in the cloud, make a plan for what to do with the data in the cloud. Maybe they put data into a data bucket. Maybe take it into a data warehouse. Maybe use a Lambda to run code, and then do machine learning.
14:11
Don: The problem is that when they need to figure out where to get data, it all falls apart. They don't have a clear path to get OT data up to the cloud.
14:22
Don: Actually, the problem is, we're taking IT solutions and trying to apply them to the OT world. It's not working. We need to turn it around. Arlen, you mentioned that. We need to turn it around and apply OT solutions to the IT world.
14:34
Don: Also, we need to decouple our devices from applications. We can't access most of our device data because it is directly coupled to a single app. Instead, we need to connect devices to infrastructure, and we need a single source of truth for all tagged data.
14:52
Don: To properly start a digital transformation journey, we need an OT edge platform, really, that first and foremost can create a better OT infrastructure. Instead of just doing coding on operating systems, we need to be able to use tools on top of a superior OT platform.

Ignition + MQTT
15:11
Don: Now, it is true that I'm mildly biased in this particular arena, however the fact is, we mentioned earlier, Ignition is an industrial application that's totally capable of doing what we’re talking about. Unlike other SCADA and ICS software, it was built to be open, interoperable and standards based.
15:29
Don: The Open Process Automation Form, or OPAF as it's called, is advocating for justly this. It was started by Don Bartusiak of Exxon took the lead with other large companies. They now have, I think a hundred organizations participating, and they want systems that are open, interoperable, standards based and secure. Because that's the only kind of system that can serve their needs now, and in the future.
15:55
Don: Ignition gives you a platform that lets you position your company for digital transformation. And for converging OT and IT because it's a superior OT solution, and it leverages proven IT technologies. Frankly, that one bullet point of unlimited licensing is very important for any kind of digital transformation endeavor. Because, you are not going to scale out and connect thousands of PLCs, bring in all that data from hundreds of thousands of sensors under traditional pricing models. Frankly, you'll break the bank, and the projects won't see the light of day that'll be stuck in some CFO's pending basket somewhere.
16:35
Don: Ignition also, is the first industrial platform to fully integrate the MQTT protocol. MQTT has become the de facto standard messaging protocol for IoT. It enables enterprises to build IIoT, and digital transformation solutions in Ignition. And MQTT has some pretty unique benefits. One, to open standard, the work done by Cirrus Link Solutions on the Sparkplug that Arlen mentioned, that project has been moved into the Eclipse Foundation for stewardship. It's the Tahu project, and it's going to continue to move forward with that open standard.
17:13
Don: It decouples also, MQTT decouples devices from applications. It allows multiple business applications to get data without affecting operations. It's a published subscribed protocol, compared to poll response protocols. These are much pub scribes much more scalable and efficient. It allows applications to connect to middle ware. It provides a single source of truth for tagged information, which incidentally will save you thousands of hours of work, and gives everyone in the enterprise a common point of verifiably correct data to work with, which I'll think you see today from both an MES view point is going to be extremely important to have that verifiable source of correct data. It's bidirectional, it has stateful awareness. Requires little bandwidth. It's really got plug and play functionality. It gives you the chance to auto discover tags. There's a whole host of other qualities of MQTT, but in the introduction, you might have noticed that one of Arlen's points is he's co-inventor of MQTT.
18:23
Don: By leveraging MQTT on the Ignition platform, you can decouple devices from applications. You can push pull into the edge of the network, and you can make data instantly accessible to that entire enterprise. But, Arlen, I really want to turn it over to you as co-inventor of MQTT and ask you to elaborate about how is this combination of benefits bringing Ignition and MQTT together, with the decoupling that it provides? How is that such a critical component to accomplishing digital transformation?
18:54
Arlen: Well Don, as I said, we've got this mindset of how we build out SCADA systems, operational systems. I would challenge everybody, if I had the Men in Black magic mind eraser, and I clicked it, and everybody forgot about poll-response protocols. They forgot Allen Bradley, they forgot Modbus, DMP 3.0, and we can start from scratch. We would leverage TCP IP, we leverage all of the capabilities that we have now, with high performance CPUs, even at the edge, and we would connect those devices into infrastructure.
19:32
Arlen: In this diagram, that's exactly what we're doing. Imagine, one of my favorite is a flow computer. In a flow computer, it's very dyslexic in that one side of a flow computer is a cash register and it goes to certain data consumers in the enterprise. The other side of the flow computer provides very important process variables for operations. Typically, one application will be polling for one set of data, another application set will be polling for another set. Imagine a flow computer that could come up, connect to infrastructure, and start publishing all the things that a flow computer knows that it's got information on.
20:11
Arlen: Now, applications can plug into that infrastructure and one application could just subscribe to, say, the daily flow information. Another application can subscribe to the roll time process variables.

20:26
Arlen: In all of the engagements that we've got now with Ignition customers, both in plant floor, in manufacturing, in oil and gas, water and waste water, is that they're finding out that now that they've got devices connected to infrastructure, and with Ignition Gateway, now they can start building out their dashboards and all of their operational aspects. But then we can plug in other applications, so for example, OSI PI can plug in, and it can auto discover those tags with all of its metrics, and all the information. Canary Labs has a historian that can do the same thing.
21:03
Arlen: We're really starting to see this ecosystem build out. We've been working with, I believe over 35 OEMs that are building these devices that will be able to plug into this MQTT architecture and then we can automatically discover tags, know their engineering units, their tag name, their engineering ranges, and if anything changes we've got one too many architectures. So, if my MES system is subscribed to that, if my cloud infrastructure is subscribed to that, if my Ignition Gateway is subscribed to that, and I change one aspect of a process variable. Say I change pressure from PSI to kilo pascals, then everybody in the enterprise is immediately notified, i.e. that single source of truth.
21:50
Don: That's great, Arlen. I really appreciate you expanding on this particular area. You mentioned the architecture for digital transformation and why this is so important. Let's move into that.

Digital Transformation Architecture Overview
22:01
Don: We're talking about digital transformation now, so we better look at an architecture overview that gets us there and gives us a better idea of what's possible.
22:10
Don: Let's take a look at this, high-level overview, and Ignition architecture that enables digital transformation, as I said. We start down on the plant floor with the PLCs and other equipment. So, Kent, I'd like to turn it over to you to sort of briefly describe the solution we could set up at this level.
22:26
Kent: Yeah, thank you Don. With this, it's hard to say, "All right, now there's all this new equipment out there that supports MQTT out of the box that you can just plug in to your infrastructure, so for all your new projects you need to go buy all new equipment." I know that, that's not always feasible. In fact, nine times out of ten that's not the plan, that's not feasible.
22:49
Kent: And so, what you end up with is you have older equipment that still talks all these polling protocols, whether it's Allen Bradley, or Modbus, or any of these other ones. And so the question is, how can I still take advantage of digital transformation without ripping or replacing everything I've already done. The answer is that you can utilize something like an Edge Gateway where you put out a small software install in an industrial PC or it can be a dedicated hardware that can connect to your existing equipment, talk to or over its existing protocols, and then poll that data into this Edge Gateway as tags and all the other stuff that you're used to configuring but then from there it can publish it into this MQTT architecture. It allows you to keep the old and still take advantage of the new. With that, Arlen, I want to pass it back to you to kind of talk about that shift of getting out old data and then how that fits in to a larger architecture.
23:53
Arlen: Okay, thanks Kent. With the Ignition Edge product, so you've got, if you know Ignition Gateway, Ignition Edge has all of the features that you're used to. You've got all of your tag management tools, you've got your protocols. If I was at the Edge and I had this Edge Gateway connected to a Modbus PLC, and I'm connected locally now, I don't have to worry about network bandwidth, I'm probably a foot away from that PLC. Now, I can pull with high speed, get all of the registers that I'm interested in, into that Ignition Edge Gateway.
24:29
Arlen: Now that I got those tags, I can start adding that single source of truth because in Ignition I can edit that tag, I can add a tag name that makes sense. I can give it engineering ranges, I can give it engineering units, I can give it a description. Now, those tags, now that they're available in that Edge Gateway are available for MQTT transmission. To publish those, connect to the MQTT server, or multiple MQTT servers and publish that information in real time.
25:00
Arlen: Now, what's happening there is that since MQTT is a stateful protocol, we publish everything on the initial connection, all of your process variable of values, but then we don't have to publish them again until they change. Everything can be report by exception, so in typical installations where we're replacing a poll response protocol with Edge and MQTT, we're looking at anywhere from an 80 to 95% bandwidth reduction, for the same about of data with faster update rates.
25:35
Arlen: Now, once we've got that Edge out there, one of the other advantages is that we've got store and forward. Tom to will talk about this later, but it's very important that if our network infrastructure went down, that we're able to store all of the tags on the Edge, with their proper millisecond timestamp, when that connection is reestablished, we're able to publish all those tags that go directly into the tag historian Ignition.
26:05
Arlen: Even with network outages, you still have 100% coverage on all of your information from the Edge, or the factory floor into your Enterprise Gateway infrastructure.
26:17
Arlen: Now, that we've got all of those tags in there, and now we're able to start accessing more information that we were leaving stranded, I'll turn it over to Tom and he'll explain what else what we can do with that information now that we've got it in our Ignition Gateway.
26:32
Tom: Thank you Arlen. To really gain insight we're going to use the information that Arlen's talking about getting up into the Gateway, and we want to gain insight to our manufacturing, but it's not as simple as just feeding time-series values for hundred thousand, ten thousand, or hundred thousand or more sensors out there into machine learning and into the MES system to provide insight into our processes.
27:02
Tom: I mean, one, it requires data to be clean. That's a very important, and that's a big part of doing MES or machine learning. It also requires context, things like the product being run, or the shift, or the operator, or the maintenance crew. I mean, you can just go on and on.
27:29
Tom: Typically, this information does not reside in the sensors, or PLCs, or other devices or other devices out there in the field. You can sometimes you see when they manually select the product they're running or what have you. Typically, it doesn't reside in those. It does however reside in ERP systems and MES systems.

27:52
Tom: The one important thing too is that MES systems, the data is clean, because you're running your production based on the MES system, and if you have invalid data then you're producing invalid products, you're not hitting your target production count. Your short shipping possibly, or you're over producing, all those kinds of things can happen if you don't have accurate data.
28:21
Tom: The MES systems, typically that data is clean. For non-sensor data, like I mentioned, it can be entered manually, but typically that leads to more time for the operator to make those selections or enter that data and it also, more mistake proof, more mistakes than typos and things like that.
28:50
Tom: It's very, very valuable to pick up this data automatically. Both from the plant floor, and pick up from legacy systems, like Kent talked about. You can pick up from new systems using MQTT, and also the store and forward that Arlen was talking about. So, MQTT is just a really good solution for getting that data in. It's not the only solution, it is the most current and most efficient way to bring that data in.
29:24
Tom: But it's also valuable to get information automatically from ERP systems or other business systems. For example, production schedules, or material definitions. Why maintain two copies of that information? You're just going to have errors and mistakes synchronizing the two of those. Why not pick that up automatically?
29:49
Tom: To do that, basically we have a Business Connector. The Business Connector allows us to provide some business logic and some transformation and mapping of data. It features a direct interface to SAP, and you can interface to other systems using web services either SOAP or REST.
30:16
Tom: You will see more direct connectors in the future. We're getting requests in for those, we're tallying that up. We're going to be expanding that as well. It also allows you to provide interfacing with cloud services a lot of them that are out there as well.
30:37
Tom: It greatly reduces the effort. Arlen and Don both talked about the amount of effort in code that you have to do when you're collecting data. Well, if it's all in code, it's really not maintainable. It's hard to trouble shoot if there's a problem, and so the business connectors is a visual interface, and basically eliminates code. It makes it a lot more straightforward.
31:07
Tom: The other thing too, when you're doing machine learning and digital transformation, and trying to get insight into your production data, there's a lot that MES can provide as well. There's a lot of KPIs that are produced, like OEE that can be used to train against. For example, what are the combinations of features, or operating conditions that result in a higher OEE. What are the combinations that result in a lower OEE? So that OEE in that case is the training label.
31:46
Tom: MES can definitely provide additional calculated data that helps in this digital transformation world. With that I'm going to pass it back to Arlen, he's going to discuss Injector Module.
32:05
Arlen: Okay, thanks Tom. Great. Let's step back here, and let's imagine, let's go from this simple diagram, and Don knows that one of the customers that we're working with when we first met him, he's in charge of digital transformation, his vision was, "Arlen, I should be able to walk into any one of 60 factories in the world that we have with proper authentication, plug my laptop into the infrastructure and in a matter of seconds learn that entire plant. Every machine, every process variable, all their engineering units, all their engineering ranges."
32:40
Arlen: Indeed, we have done that. So we've got a demo where we show 1,400 PLCs with 60,000 tags connected into MQTT infrastructure, enable MQTT Engine and in less than 10 seconds we have all 60,000 of those tags in our Ignition Gateway, with all their definitions.
33:02
Arlen: Now, our MES can start leveraging all that tag information. We've got storing forward, so we don't have to even worry about data outages. But now that we've got that information we can do even more things with it. One the things we've have several customers today, is they're creating, since they have several machines and manufacturing that work together. In their Ignition Gateway, they're able to take those tags and put them together and build this very overused term of a digital twin. And now, I've got that digital twin tag in my Ignition Gateway, and I want to get that up to some machine learning.
33:40
Arlen: With our Injector modules, with the four versions of Injector, you can take any tag, or all the tags that are in your Ignition Gateway, and be able to publish those into the typical ingest engines on IBM Watson, Azure IoT, AWS IoT using Kinesis screens, Kinesis Firehose, or DynamoDB or into Google Cloud platform.
34:06
Arlen: Now, the other interesting point here is that the same Sparkplug specification that let me do automatic learning of all those tags in Ignition is used to represent those tags when they're published up to the cloud, and then when they're put into something like a data lake. So, if you think about that, instead of writing code in a Lambda to know what topic to subscribe to on your AWS Greengrass Core, now you've got a common topic name space, a common data representation from your equipment in the field into your operational system. Now, we've done what Don and I were talking about, let's build this, let's build a better OT system but now we're able to leverage into the cloud. Instead of me trying to start in the cloud and look down and go, "Oh, that's a mess." No, let me put together an infrastructure that is operationally better, that is already cloud friendly. It already enables us to get that information in context into the cloud.
35:16
Arlen: Once we have the information up there, I'm going to turn it over to Kathy to explain what we can do with it.
35:22
Kathy: Thanks, Arlen. We've seen the pieces of a machine learning project. First, I want to review the workflow a little bit. As Don said earlier, you need to pick a problem that meets OT's needs, and has real business value. You want to be sure that the prediction you make will allow you to take an action, such as changing equipment settings or scheduling employees. Because a prediction without an action, that's really cool but there's very little ROI on that.
35:50
Kathy: Now, that you have a project you need data to train your model with. The Ignition historian and the Sepasoft Business Connector are good sources of historical data, but you should count on spending about 70% of your project time collecting enough data and cleaning that data for your modules.
36:05
Kathy: As Tom pointed out, if you've been using MES, your data's probably pretty clean, but likely you're going to need to pull in some data from other sources, or from before the time you started using those modules. Do count on spending the time. Don't skimp because the quality of your model depends on how good your data is.
36:26
Kathy: The Cirrus Link Injectors will get your data to one of several different cloud-based machine learning systems, and there are a couple of big advantages to using cloud-based systems. One is that you can scale up and down instantly. Initial training of machine learning models can take a lot of computing power. That flexibility is very important to your project.
36:46
Kathy: Another big advantage is cloud-based systems have a lot of algorithms to choose from. Even experts have to try several algorithms to find the best one for a particular project. Being able to switch with a couple of mouse clicks, will make it easier to find the right model for you.
37:02
Kathy: Part of picking the right model is scoring that model, which simply means finding out how accurate your predictions are. Decide beforehand what level of accuracy you need. Is doing a little better than guessing good enough for you? Or, are mistakes so costly that you need 99.99% accuracy. More accuracy will come at a price. A good rule of thumb is adding 1/9 to your accuracy, is going to increase your project cost by about one hundred times.
37:29
Kathy: Once you've trained and scored your model, you can either keep your model in the cloud and let Ignition query the model for new predictions, or you can use something like Greengrass to bring that model back to your edge of network and have Ignition query it locally. Which way you go will depend on your unique needs.

37:45
Kathy: One final piece of advice is don't be afraid to iterate. A good machine learning project that provides real value is worth the time. With pieces like Ignition, the business connector, and cloud connectors in place, making improvements to your project will go a whole lot smoother.
38:00
Kathy: And now I'll turn it to Tom and he'll show us a demo of what's possible.
38:04
Tom: Thank you, Kathy. We're looking at the Ignition Designer here. I could do this in the client, but there's some things I want to show in the Designer, so we'll just stay in the Designer here. It's just a real simple example, but it's covering all the different points that we're talking about here to do prediction.
38:29
Tom: We're making a selection of the operator here and product code, and we have in our system, our MES system, we have a target rate for that product code. But, we're going to go out, and we're going to get a predication of what the actual production rate will be. I'll describe the benefits of that in a little bit here.
38:50
Tom: So, we're going to get that prediction from Amazon SageMaker, which is up in the cloud, which is a machine learning platform that you can use to train and create models to get predictions from.
39:04
Tom: We're going to communicate to Amazon SageMaker using the business connector and the web services module. Let me just select an operator here, select a product. I'm going to go ahead and start a run, and we see our target rate was 120, but it's predicting that we can expect based on our historical data that we used to train, we can expect 116, just over that.
39:38
Tom: This becomes valuable because this is going to tell us we're probably going to go past our scheduled time for that production run. We can start making plans for that. If it's other things that follow behind it, we can make adjustments, other production runs and stuff.
39:57
Tom: Let me get some other data in there, put something else here. Let me get one more here. Okay. Here, our target rate is 100, and its actually our predicted rate is over that. So now we can make plans and start staging the next product that we need to run, get the materials to line, or what have you in advance, and we don't have a delay where we're waiting for materials because we've finished up early.
40:32
Tom: It really becomes this valuable information. And this is just a simple example, but this is what the types of things that you can do with that. I'm going to go over now to SageMaker here. This is the environment where you have these notebook instances, where you create your model and you can do your training of your model, and stuff like that.
41:01
Tom: We have our model down here, and our model is basically the mathematical information that we can now calculate our predictions with. And then we have an endpoint. This is going to expose this model to us, so that we can access it through the business connector, or other systems, actually. Not just the business connector.
41:31
Tom: It gives us a URL and we can make the request up to Amazon SageMaker to get our predictions. As Kathy mentioned, you have Greengrass where you can put that, once you've trained it, you can put it in Ignition. Ignition is completely capable of that. Depending on your situation, you might want to just make the calls up in to it. That's what this demonstrating.
41:58
Tom: The first step we'll take a look at is the web service, and we have a restful configuration. The consumer, and basically we have our URL there. We have our data going in and our prediction coming back. Our request is the data and our response is the prediction. So that's going to actually handle the protocol of going to Amazon, saying give me the prediction, and getting that result back. But we have to be able to handle that and process that.
42:33
Tom: Now we come over to the Business Connector. The Business Connector has a data flow sequence editor here. It can get real complicated where you go to ERP system and request material list, and then iterate through those. Get the material details, add them to MES, all that kind of stuff. This is just a real simple sequence. We're going to just make our request up and return the prediction.
43:00
Tom: You can see where SAP connector is here, as well, RESTful, and SOAP, and other items are in here. This chart, as we call it, we have parameters. We're going to pass in the product code, operator, and target rate. Those were the things that we saw in our example screen, and we're going to return back the predicted rate.
43:26
Tom: Then we go and we're going to make our RESTful call, we're selecting this web service configuration there. We have to pass our parameters in. So we have our operator, our product code, and our target rate, all passing in to the data. And you can drag and drop and map these very simply. I'm not going to get into that too much. That's passing our data in before we make the RESTful call. And then afterwards, we get back our prediction. And we're going to put that over into our predicted rate parameter.
44:13
Tom: You saw no code there. We didn't have to do any scripting or anything like that. I am going to go to the script console here that's in Ignition. It allows me to execute this chart, and give you an idea of how you invoke this. I have the input parameters, and I just hard coded some values, so in the sample screen I did first, those were dynamic and I was putting them in. And then we're executing that chart, and this is the chart we're executing. There's our input parameters, and I want to get the predicted rate back.
44:49
Tom: If I go ahead and execute that, there's my predicted rate for these parameters. So really that's all there's to it. I have this small amount of script over here when I make these selections. That's how it's going out and getting that predicted rate. So pretty streamlined and simple.
45:15
Tom: Now, that information and this predicted rate we can utilize that over an analysis, and start putting it on charts, making dashboards with it, including it in reports. This is an impromptu analysis here to where you can just make those selections up here and include that in what you want to look at.
45:40
Tom: So I hope this kind of demonstrates all the way from the field devices, getting the data into Ignition here, I didn't cover that in detail, but then also what we do with that in MES, and how we get a prediction in MES. All the little-known mechanics and tools of from plant-floor field devices, all the way up to Amazon SageMaker and back is kind of covered there without too much complexity.
46:12
Tom: I will pass that back to Don now for a recap.

Closing Thoughts on Achieving Digital Transformation
46:16
Don: Thanks a lot, Tom. I think you can see from the demo really that the whole point we're trying to make here is just the digital transformation is within reach, if you go the right approach, the right platform and the right tools to work with.
46:28
Don: Just a quick recap, I'll ask each of our co presenters to give any final thoughts they have, and then we'll move into a Q&A.
46:35
Don: Digital transformation is having a profound impact. Many businesses are starting to realize its full potential. IT led digital transformation efforts aren't working. To realize the promise of digital transformation companies need a stronger OT platform that they can build on.
46:52
Don: With Ignition and MES, MQTT and Cloud Injector Modules you can have a better OT platform and connect your OT data to the cloud, to do what you want with it. So then it opens up machine learning, can be sent from the cloud back to the equipment level fulfilling the promise of digital transformation.
47:10
Don: Any thoughts of what companies need to achieve digital transformation, what they're looking at? Kathy, let's start with you.
47:17
Kathy: The one thing I would say is give yourself enough time to do the project properly. This is not something that you're going to knock out in an afternoon. To really get the return that you're looking for, allow plenty of time to properly clean your data, and try different models.
47:33
Don: Great, thanks. Kent?
47:35
Kent: Yeah, and from a realistic standpoint here, when people are looking to implement digital transformation, I've seen a lot of companies successfully put data in the cloud, and then they're not doing anything with it. But they feel like, "Check. I did it. I am successful, data is in the cloud."

47:52
Kent: I would hope that we've covered a little bit today that when we're getting data to the cloud, you should be adding context to that data, you should be heading there with a goal in mind, that you have a specific ROI you're looking at. Whether that's like in the example that Tom just showed us, whether that's trying to predict production, or whether you have your own maintenance cycle that you're trying to optimize or something like that. Going with a goal so that a completed project has value, not just a check mark that you got data somewhere different.
48:26
Don: Thanks, Kent. Tom, your final thoughts?
48:29
Tom: Yes, well I can definitely echo what Kathy and Kent are saying there, and that's what I've seen in my experience. I think that for the manufacturing type of things, MES is kind of like a starting point. It's a vital part of it. If you're recording information on paper currently, who's the operator, what's the product code, those kinds of things. You're not going to be able to do this very effectively. MES is an essential part of that.
49:02
Tom: Now, if you get into other areas, processes and other things that maybe you don't need that context, then maybe MES doesn't apply, but in manufacturing, I think it definitely does. Thank you.
49:17
Don: Thanks. Arlen?
49:20
Arlen: Again, I'll agree with what everybody has said, is that we indeed have lots of injector modules out there, we have lots of data going into the cloud, and lots of data just sitting there. So, again, we've said this before, instead of trying to eat the elephant, I think with what we've shown here, with tools on platforms, not having to write code, not having to develop a lot of Lambdas, and having tools that let me enable technicians to configure things in the field the way that they're used to doing it with typical SCADA, but then coming up with an architecture where now I can focus on one thing, and I can go to multiple cloud service providers, like Kathy said, each one of them have strengths and benefits that we should be able to leverage. We should be able to switch back and forth between those very, very quickly. So I think the whole thing here is that concept of Ignition as a platform giving you the tools that you need for OT-to-IT digital transformation.
50:27-56:06
(The speakers use the remaining time to answer attendees’ questions. The Q&A portion can be heard in the webinar video at the top of the page.)
Want to stay up-to-date with us?
Sign up for our weekly News Feed.